Matrix Design: Understanding Structure and Applications
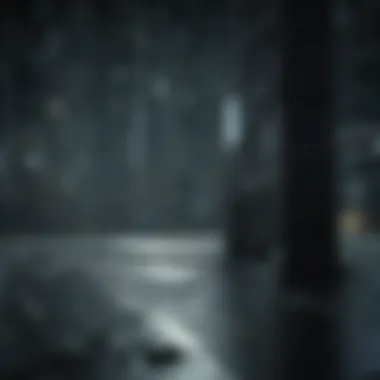
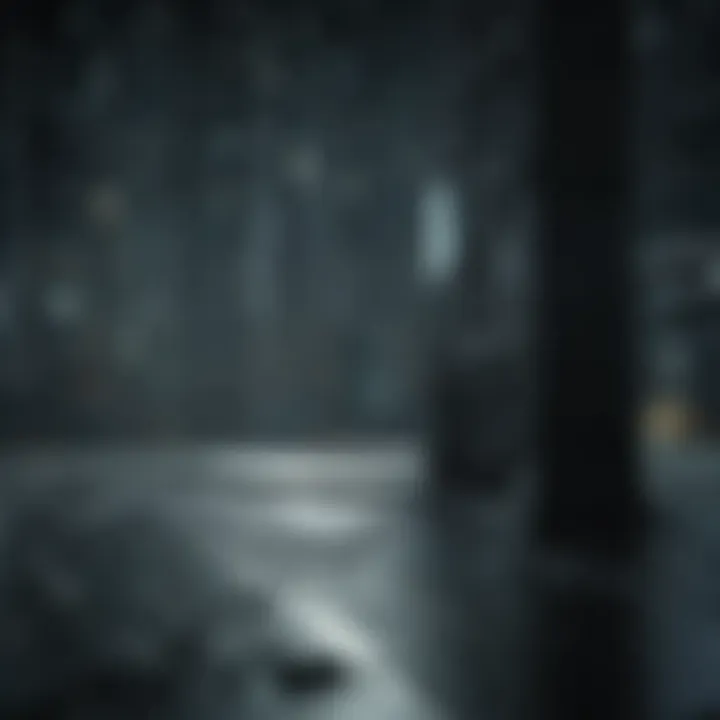
Intro
Matrix design is a vital concept in various scientific disciplines, serving as a framework for organizing information, analyzing data, and conducting experiments. The effectiveness of matrix design is not limited to the scientific method but extends to pedagogical strategies that facilitate learning and understanding. In exploring this topic, it is essential to unpack its structure, applications, and implications in a clear and systematic manner, providing valuable insights for students, researchers, educators, and professionals alike.
Background and Context
Overview of the Research Topic
Matrix design involves arranging data into a structured format, often represented as grids or matrices. This method aids in comparison, analysis, and visualization of complex information. It provides a systematic approach to manage variables and outcomes in research, enabling clearer interpretations and conclusions. The significance of matrix design stretches across multiple scientific fields, including psychology, biology, and education, influencing how knowledge is constructed and understood.
Historical Significance
The development of matrix design can be traced back to early studies in statistics and experimental design. As researchers sought to better understand relationships among variables, the matrix format emerged as a preferred method. Landmark studies in the mid-20th century established the foundation for contemporary applications. Notably, the advent of computers further revolutionized data analysis, allowing researchers to utilize matrices for complex calculations and visualizations. This historical context lays the groundwork for understanding current applications and challenges in matrix design.
"Matrix designs offer a unique lens through which researchers can comprehend and interpret data in innovative ways."
Key Findings and Discussion
Major Results of the Study
The exploration of matrix design reveals significant advantages, including enhanced clarity in data presentation, greater efficiency in analysis, and improved collaborative efforts within research teams. In educational contexts, matrix design facilitates better learning outcomes by providing structured frameworks for information assimilation. The findings indicate that students engage more effectively with material presented in matrix formats, reinforcing the need for its adoption in teaching methodologies.
Detailed Analysis of Findings
- Data Organization: Matrix design simplifies complex data sets. This clarity allows for quicker identification of patterns and anomalies.
- Enhanced Communication: Through visual representation, matrix design aids in conveying intricate ideas, making it easier for diverse audiences to engage with scientific content.
- Research Collaboration: The use of matrix frameworks can enhance team dynamics, fostering discussions grounded in visual data representations, promoting shared understanding.
- Pedagogical Benefits: Educators report improved student retention and comprehension when utilizing matrix design in lesson plans.
Intro to Matrix Design
Matrix design is a crucial framework that influences how data is organized, analyzed, and interpreted across various scientific domains. It provides a clear structure that enables researchers and practitioners to engage with complex data sets systematically. By adopting a matrix design approach, one can better handle large volumes of information, ensuring clarity and coherence in presenting findings. The benefits of understanding and applying this concept span multiple disciplines, making matrix design relevant not only to data-centric fields but also to education and research methodologies.
This article explores several dimensions of matrix design, including its definitions, historical context, theoretical frameworks, applications in diverse scientific sectors, key considerations for effective design, and emerging future directions. Each section presents insights that contribute to an understanding of how matrix design shapes research and educational practices. Thus, the importance of matrix design cannot be overstated; it serves as a foundation for logical analysis and effective communication of research results.
Defining Matrix Design
Matrix design refers to an organized approach for structuring data where elements are represented in rows and columns. This arrangement allows for ease of access and analysis of variables. A matrix can be a mathematical structure, a data table, or a model that captures relationships among variables. In essence, it simplifies the complexity of data by providing a visual representation, which is easier to interpret than unstructured text.
Furthermore, matrix design is not limited to numerical data; it can also incorporate qualitative data, making it versatile. For instance, in biological research, a matrix may represent gene expression levels across different conditions.
Historical Context
The concept of matrix design has roots in various scientific fields. It can be traced back to early mathematical theories in linear algebra, where matrices were used to solve systems of equations. The use of matrices in this mathematical context laid a foundation for their application in statistics and computer science. As data began to proliferate in the twentieth century, the need for efficient organization methods emerged. Researchers developed matrix designs to help with data organization, especially in fields such as psychology and biology.
Over the decades, matrix design has evolved significantly, adapting to the changing landscapes of technology and research methodologies. With the advent of computer science and data analysis software, matrix designs have become more sophisticated, facilitating advanced analytics and visualizations. This historical evolution aligns with the increasing complexity of the data that researchers handle today, further underscoring the ongoing relevance of matrix design.
Theoretical Frameworks of Matrix Design
The domain of matrix design is underpinned by various theoretical frameworks that shape its application across disciplines. These frameworks serve as the foundation for not only understanding matrix design but also applying it effectively in real-world scenarios. By exploring the mathematical principles and practical implementations, we can appreciate the versatility and the nuances involved in creating effective matrix designs.
Mathematical Foundations
Mathematical foundations are crucial in matrix design. At the core, matrices are structures that can represent data in a compact form. They allow for operations such as addition, multiplication, and transformation, which are vital in many applications. The linear algebra that governs these operations provides a language for scientists and researchers to formulate problems, run simulations, and analyze results in a structured way.
A matrix can be defined as an arrangement of numbers in rows and columns. Each element within the matrix can represent various attributes depending on the context. For example, in a biological study, a biomarker could be represented as an entry in a matrix, with different samples forming the rows and various measured variables forming the columns. This organization not only saves space but enhances clarity.
When dealing with large datasets, computational efficiency is paramount. Operations on matrices can be performed simultaneously, leveraging vectors and matrices to improve speed. This is particularly advantageous in fields such as machine learning and data analysis, where extensive calculations are necessary. Thus, a solid understanding of the mathematical principles is essential for effectively utilizing matrix design.
"Understanding the underlying mathematics of matrix design is key to unlocking its potential in various fields of research."
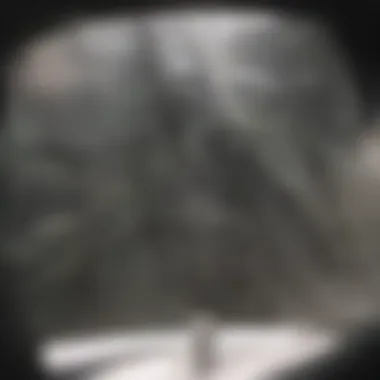
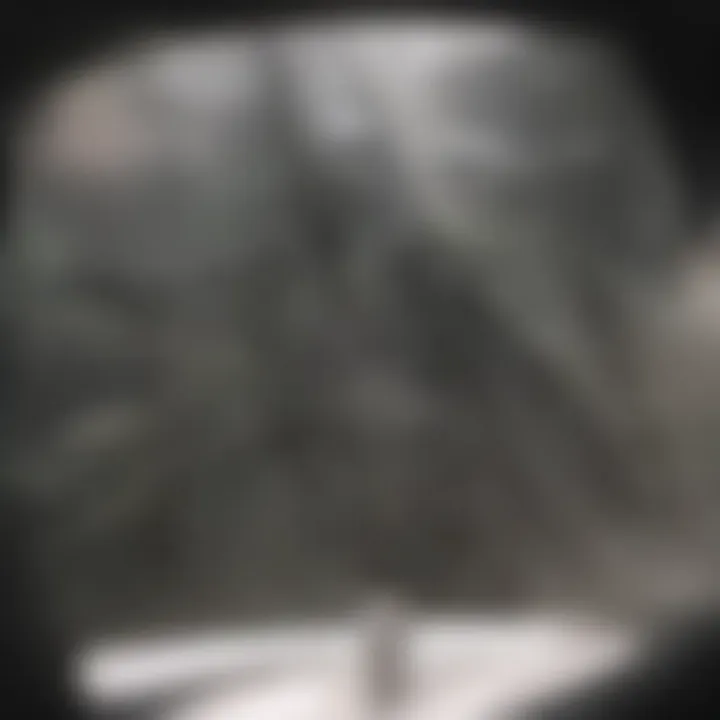
Practical Implementations
The practical implementations of matrix design manifest in different settings, reflecting its adaptability and relevance. In biological research, matrices can be used for gene expression analysis, where each row represents a different gene and columns represent various conditions or treatments. Such matrix designs facilitate comparative studies and can help answer complicated biological questions through manageable structures.
In the realm of chemistry, matrix approaches can assist in the organization of experimental data. For instance, researchers might categorize the effects of different solvents on reactions in a matrix format. This layout allows clear identification of patterns and relationships among the variables.
In engineering, matrices play a role in simulations, particularly in structural analysis and fluid dynamics. Engineers can model complex systems and evaluate responses to various loads and conditions by using matrix equations. Moreover, in social sciences, matrices help in surveys and data collection, where responses can be represented in a systematic manner, aiding statistical analysis.
To summarize, the mathematical and practical frameworks underpinning matrix design are essential for its effective use across disciplines. Understanding these frameworks allows for better implementation and application, contributing to advancements in research and analysis.
Applications of Matrix Design in Various Fields
The applications of matrix design span numerous disciplines, each utilizing this approach to enhance understanding, execution, and outcomes. Matrix design serves as a versatile framework, facilitating effective organization of data and processes while also fostering interdisciplinary collaboration. In this section, we explore the significance of matrix design across various domains including biological research, chemistry, physics, engineering, and social sciences.
Matrix Design in Biological Research
Matrix design in biological research is pivotal for structuring complex biological data. Researchers often deal with vast datasets comprising genomic, proteomic, and metabolomic information. The employment of matrix design allows for clarity in the analysis of relationships among biological entities.
- Data Structuring: By organizing data in a matrix format, researchers can efficiently identify trends and correlations. For instance, gene expression data often takes a matrix form where rows represent genes and columns denote experimental conditions.
- Facilitating Experiments: Matrix designs are often paired with experimental methodologies such as factorial designs, enhancing the understanding of biological responses under varied conditions.
">Matrix design serves as a foundation for biological research, guiding effective data interpretation and advancing discovery."
Matrix Design in Chemistry
In chemistry, matrix design finds its application primarily in experimental setups and theoretical modeling. By employing matrices, chemists can represent and manipulate quantities, structures, and transformations.
- Reaction Pathways: Matrix design aids in delineating pathways in chemical reactions, enhancing researchers' understanding of the sequence of reactions and intermediate compounds.
- Spectroscopy and Analysis: In areas such as NMR and mass spectrometry, data can be organized in matrix formats to better analyze chemical compositions, revealing valuable insights into molecular structures.
Matrix Design in Physics and Engineering
Physics and engineering utilize matrix design for analyzing systems and designing engineering solutions. This structured approach allows for better handling of complex systems involving multiple variables.
- Modeling Dynamic Systems: In physics, matrices are used to describe systems of linear equations, particularly in mechanics and fluid dynamics. This enables the simulation of changes over time under different forces and conditions.
- Structural Engineering: Engineers apply matrix design when analyzing forces on structures. By creating matrices that map loads, they can predict failure points and optimize designs for safety and efficiency.
Matrix Design in Social Sciences
The application of matrix design within the social sciences can profoundly impact research techniques and data analysis. By employing matrices, researchers can better analyze complex social phenomena.
- Survey Data Analysis: Matrix design helps organize survey responses, where rows signify respondents and columns represent different questions. This structure aids in identifying patterns and correlations in human behavior.
- Social Network Analysis: In social sciences, matrices assist in understanding relationships and influences within communities. By analyzing interconnectedness among individuals, researchers can gain insights into social dynamics and behaviors.
In summary, the application of matrix design across various fields not only simplifies data management but also enhances analytical capabilities, making it a fundamental tool in contemporary research and practice.
Designing a Matrix: Key Considerations
Designing a matrix is not a trivial task. It requires careful planning and attention to detail. The structure of a matrix directly influences its effectiveness in organizing and presenting data. Several key considerations must be taken into account in order to ensure that the matrix serves its intended purpose. Understanding these elements can greatly enhance research methodologies, facilitate data analysis, and improve pedagogical strategies.
One must consider the following elements when creating a matrix:
Elements of Effective Matrix Design
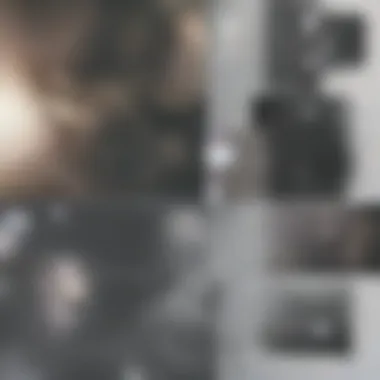
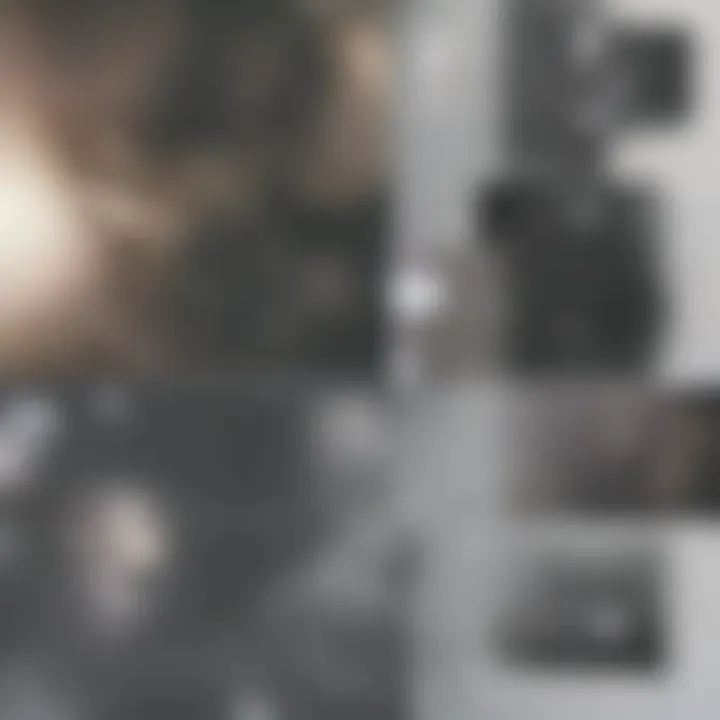
- Clarity of Purpose: Define what the matrix aims to achieve. Each matrix should have a clear objective aligning with the research needs or educational goals.
- Data Organization: Group related information effectively. The organization of data in rows and columns must be logical and intuitive to aid in quick understanding.
- Scalability: Ensure the matrix can be expanded without losing coherence. A matrix should accommodate additional data points without complicating the structure.
- Usability: A matrix should be user-friendly. The design should allow users to interpret the information easily. Avoid overly complex designs that could confuse the audience.
- Aesthetic Considerations: Visual appeal matters. An intuitive layout with a clean design can improve engagement. Use color coding or shading wisely to highlight distinctions within the data.
- Flexibility: Adapt the matrix design for different contexts. It should be versatile enough to be applied across various disciplines while retaining its core elements.
Common Pitfalls in Matrix Design
Despite the clear advantages of a well-structured matrix, there are common pitfalls that designers encounter:
- Overcomplication: Adding too much information can overwhelm the audience. Keeping the matrix simple often yields better results.
- Neglecting User Feedback: Ignoring the input from potential users can lead to a design that does not meet their needs.
- Inconsistency: Maintain consistent terminology and formatting throughout the matrix. Inconsistencies can confuse users and diminish clarity.
- Lack of Context: Providing no background on how to interpret the matrix can lead to misinterpretation. Always include relevant information or guides for users.
"A well-designed matrix does not merely present data; it transforms data into insights that can drive decision-making."
- Ignoring Limitations: Every matrix has its constraints. Failing to acknowledge these constraints can lead to unrealistic expectations about the information it can convey.
Creating a matrix is both an art and a science. By considering these elements and pitfalls, one can craft a matrix that is not only functional but also insightful. This section lays the groundwork for effective matrix design, which will reverberate through its various applications, from scientific research to educational methodologies.
Challenges in Matrix Design
The utilization of matrix design presents unique hurdles that can affect its effectiveness and overall application. Understanding these challenges is crucial for researchers and practitioners who rely on this method for organizing and analyzing complex data. By addressing specific elements, one can harness the benefits of a well-constructed matrix design while mitigating its potential pitfalls.
Technical Limitations
Matrix designs can often encounter technical limitations that hinder their effectiveness. These limitations can arise from the software and tools used for matrix construction and analysis. Often, standard software may not support the complexity and size of matrices required for advanced studies. For example, popular statistical packages may struggle to handle large data sets, leading to inaccuracies or longer processing times. Additionally, some matrices may require non-standard functions, which might not be available in every tool. These technical challenges emphasize the need for selecting the right tools and verifying their capabilities to work with the specific matrix design implemented.
Another concern involves the integration of various data types within a matrix. When various data forms, such as qualitative and quantitative, are combined, data integrity can be compromised. Researchers must ensure consistent data formatting, which is a demanding task, especially in extensive datasets. Furthermore, it is crucial to understand the algorithms or analytical approaches being used. Sometimes, researchers might apply inappropriate statistical methods that lead to misleading results. Thus, it is vital to remain vigilant regarding technical requirements.
Interpreting Data from a Matrix
The interpretation of data sets structured as matrices can be challenging. Matrices can display a wealth of information, but synthesizing that information into meaningful insights requires careful analysis. The first hurdle is to ensure clarity in the definition of the matrix's variables and relationships. Misinterpretation can occur if the variables are not well understood. Consequently, the reader or analyst might derive false conclusions, which can impact research outcomes.
Moreover, the relationships among the data points must be discerned correctly. This includes understanding how rows and columns interact and how each element contributes to the overall findings. Often, stakeholders can struggle with extracting the most relevant patterns or trends from the data provided. Thus, effective training on interpreting matrix data is essential for users, particularly in a multidisciplinary context. This training can provide the knowledge necessary to discern key insights.
"Interpreting data from a matrix is not just about number. It involves understanding their connections and meaning."
In summary, to address the challenges in matrix design, one must recognize technical limitations and ensure proper interpretation of the data. Emphasizing clear definitions and robust data analysis is vital to leverage the full potential of matrix structures. These considerations are essential to maintain the integrity of research and data analysis, especially within high-level academic and professional contexts.
The Role of Matrix Design in Data Analysis
Matrix design significantly influences how data is organized and analyzed in various research fields. This structured approach allows researchers to manage vast sets of information efficiently. By organizing data within a matrix structure, clarity is achieved. Insights can be drawn more readily as relationships between variables become apparent. Moreover, the implementation of matrix design supports systematic analysis methods, ensuring that important patterns do not go unnoticed.
Organizing and Analyzing Data
The process of organizing data using a matrix design streamlines information flow from raw data to analysis. An effective matrix creates a framework where every variable can be listed ensuring that they are not overlooked.
- Clarity and Simplification: In a matrix layout, rows and columns represent different categories or variables. This organizes data neatly, which helps in avoiding confusion and errors during analysis.
- Facilitation of Computational Algorithms: Many analytical tools and software programs work better when data is structured in a matrix form. For example, in statistics, tools like R or Python allow easy manipulation and analysis when presented with matrix data.
One common example is the use of a correlation matrix, which allows researchers to quickly identify relationships between multiple variables. In experimental designs, such as in clinical trials, a matrix helps ensure that all necessary variables are considered for each participant. Thus, it optimizes research outcomes.
Visualizing Matrix Data
Visualization is a critical aspect of data analysis, and matrix design plays a crucial role in creating effective visual representations.
- Ease of Interpretation: Graphical representations like heat maps can visualize matrix data effectively. These visual tools enable scholars to quickly interpret complex datasets. For instance, a heat map can show the correlation coefficients between different variables, allowing immediate identification of patterns.
- Supporting Decision Making: Visually presenting matrix data can assist stakeholders in decision-making processes. It enables them to see trends and anomalies clearly which might remain hidden in raw data.
"An effective visualization can lead to insights not obtainable through numerical analysis alone."
The combination of organizing and visualizing data through matrix design leads to better understanding and insights in research. Together, these elements create a robust framework for effective data analysis.
Matrix Design in Education
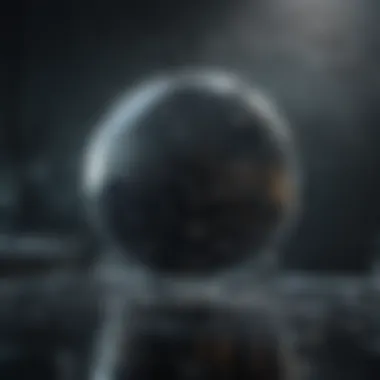
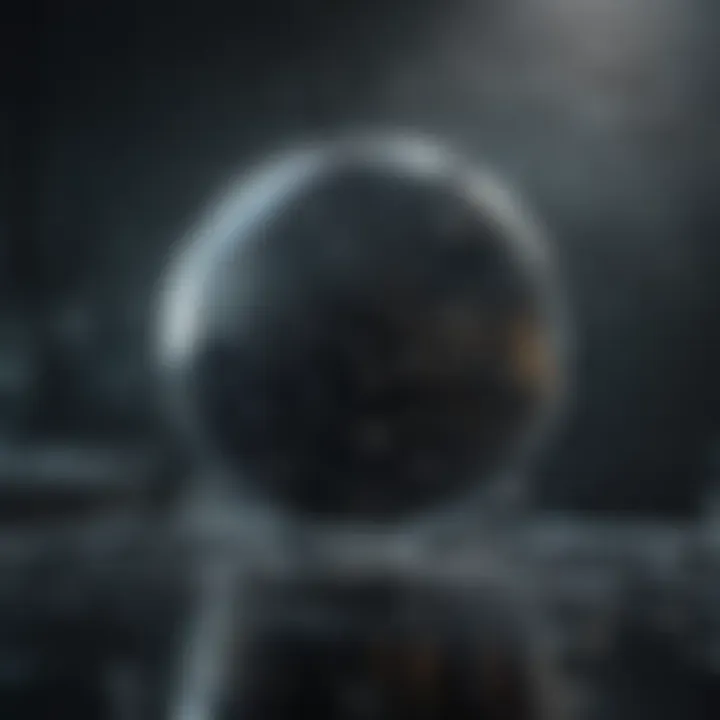
Matrix design in education plays a vital role in structuring learning environments that foster both understanding and critical thinking. It allows for an organized approach that can enhance learners' engagement with content across various subjects. With the growing emphasis on evidence-based methodologies in teaching, matrix designs enable educators to create frameworks that facilitate not just knowledge retention but also complex problem-solving skills. They can be utilized to arrange curricula, assessments, and instructional strategies effectively.
By using matrix design, educators can identify connections between different topics. This linkage can aid in creating a coherent educational experience. Through visual organization, both educators and students gain a clearer understanding of material relationships. Furthermore, matrix structures can address diverse learning needs by allowing for flexible pathways to access information.
Teaching Strategies Using Matrix Design
When implementing matrix design in educational settings, several teaching strategies can be adopted. First, educators can employ concept maps that illustrate interrelations among various topics. This approach supports students in recognizing how different ideas connect, promoting a more integrated understanding of the subject matter.
Second, project-based learning is enhanced by matrix design. By laying out goals, timelines, and roles in a matrix format, students obtain clarity on expectations and responsibilities. This approach promotes collaborative skills and encourages students to engage critically with the project objectives.
Moreover, matrix design facilitates differentiated instruction. Educators can customize learning experiences to meet studentsโ varying needs by presenting materials in tiered levels of complexity. This approach equips students with choices tailored to their interests and readiness levels, optimizing the learning experience.
Matrix designs also support formative assessments, allowing educators to effectively gauge studentsโ understanding throughout the learning process. This type of assessment can highlight specific areas where students may need additional support, ensuring timely intervention.
Developing Critical Thinking Skills
Developing critical thinking skills is fundamental in education, and matrix design can significantly contribute to this objective. By engaging in activities that require students to analyze, evaluate, and synthesize ideas, learners can sharpen their ability to think critically about complex problems.
One effective strategy is to use case studies organized in a matrix format. This method encourages students to explore multiple perspectives and draw connections between theoretical knowledge and real-world applications. As students deconstruct case scenarios, they practice logical reasoning and enhance their inquiry skills.
Discussion forums structured by matrix design can provide another avenue for promoting critical thinking. In these forums, students can systematically present arguments, elaborate on counterarguments, and evaluate differing viewpoints. This structured interaction nurtures a deeper comprehension of the subject matter and develops debate skills essential for articulate reasoning.
Ultimately, employing matrix design in education cultivates an environment where critical thinking thrives. Students become active learners, engaged in deep analysis rather than mere rote memorization.
In summary, matrix design provides educators with valuable tools to enhance teaching strategies and develop critical thinking skills. This approach holds significant potential to transform educational outcomes, promoting a more engaged and thoughtful student body.
Future Directions of Matrix Design
The future of matrix design holds significant promise as new technologies and methodologies continue to evolve. This section explores the specific elements that will shape matrix design moving forward. The integration of technology into matrix frameworks offers a range of benefits. These enhancements make data collection easier, increase accuracy, and streamline the analysis process. With these advancements, researchers can tackle more complex problems more efficiently.
Emerging Technologies and Matrix Applications
Emerging technologies are poised to redefine how matrix designs are constructed and utilized. Significant trends include the utilization of artificial intelligence and machine learning in matrix applications. These technologies enable the processing of large datasets, enhancing pattern recognition and predictive modeling. As a result, researchers can derive insights more rapidly than before.
Moreover, software platforms like R and Python libraries such as NumPy and pandas provide powerful tools for managing matrices. These tools simplify data manipulation and allow for robust statistical analysis. Collaborative technologies are also vital. They promote teamwork and data sharing across disciplines. This fosters innovation and leads to the development of cutting-edge matrix applications.
"Emerging technologies in matrix design not only enhance data analysis but also facilitate collaboration across diverse fields, enriching the research process."
Interdisciplinary Approaches
An interdisciplinary approach is crucial for realizing the full potential of matrix design. By merging insights from various fields, researchers can innovate and apply matrix concepts in novel ways. For instance, the blend of matrix design and neuroscience can lead to new insights into cognitive processes, while combining it with social sciences can help in understanding complex social networks.
Furthermore, sharing methodologies between disciplines can provide solutions to common challenges. For example, the principles of matrix design in biology can inform techniques in computational analysis in computer science, and vice versa.
As matrix design evolves, it is essential that educational institutions incorporate these interdisciplinary approaches into their curricula. By equipping students with diverse skill sets and collaboration experience, they are better prepared to tackle the challenges of tomorrow's research landscapes.
Ending
The conclusion serves as a vital overview of the key themes discussed throughout the article on matrix design. It synthesizes the knowledge gained from various sections and underscores the significance of an effective matrix design in research, education, and data analysis. While highlighting the multifaceted applications of matrix design, it emphasizes how these structures facilitate organized thought processes, improve data management, and enhance analytical capabilities across disciplines.
Summary of Key Insights
Many key insights emerge from the exploration of matrix design. Some essential points include:
- Foundation of Structure: Matrix designs provide a framework that structures complex data efficiently, allowing researchers to see patterns and relationships more clearly.
- Interdisciplinary Relevance: The applications of matrix design stretch across fields such as biology, chemistry, physics, and social sciences. Each discipline benefits uniquely from the capacity of matrix designs to organize and interpret intricate information.
- Challenges and Limitations: Despite their advantages, challenges remain. Technical limitations and difficulties in data interpretation can hinder the effectiveness of matrix designs.
- Educational Applications: In educational settings, matrix designs can enhance teaching strategies and improve critical thinking skills among students, showcasing their value in knowledge dissemination.
- Future Directions: Emerging technologies and interdisciplinary approaches signal a promising future for matrix design. The continued evolution may lead to enhanced methodologies in research and education.
Final Thoughts on Matrix Design
Matrix design is not merely a technical tool; it is a fundamental approach to structuring information. As research methodologies evolve, so too must the frameworks we utilize. The benefits of matrix design extend beyond simple organization; they foster deeper understanding, enable better decision-making, and drive innovation across various fields. It remains essential for academics and practitioners to embrace matrix design, adapting to the specific needs of their disciplines while remaining aware of inherent challenges. By doing so, we pave the way for a more informed and analytical approach to research and education.
"In a world where data is abundant, matrix design stands as a guide, navigating complexities with clarity and purpose."
The continuing study and application of matrix design will undoubtedly influence future academic inquiry and professional practice.