Exploring Mofa Multi Omics: A Comprehensive Overview
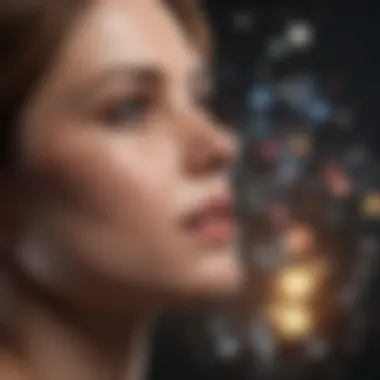
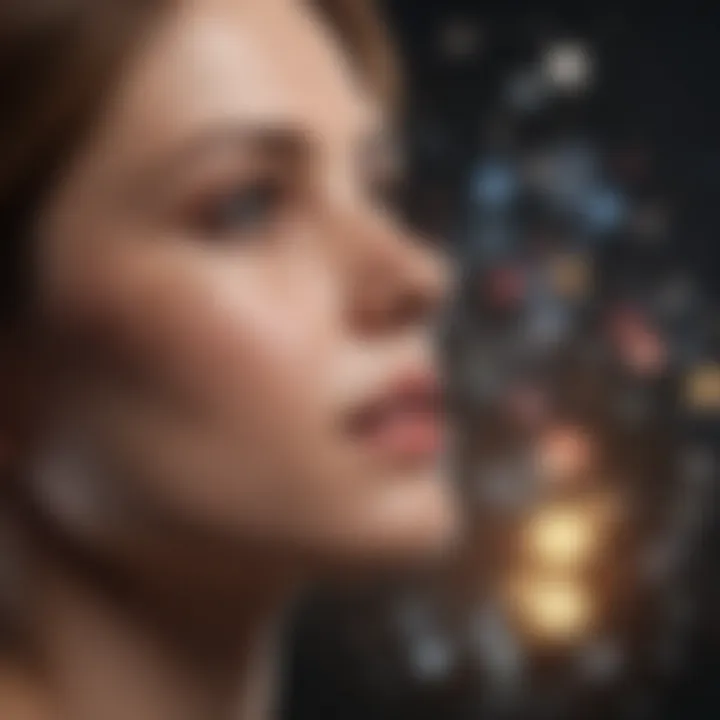
Intro
In recent years, the field of biological research has evolved rapidly. One of the most exciting developments is the rise of Mofa Multi Omics. This approach focuses on fusing various omics data types such as genomics, transcriptomics, proteomics, and metabolomics. The aim is to gain a deeper understanding of biological processes and disease mechanisms. This integration of layers is vital as it allows researchers to explore complex biological phenomena that cannot be captured with a single omic layer.
Background and Context
Overview of the research topic
Mofa Multi Omics stands at the intersection of data analysis and biological inquiry. It leverages machine learning techniques to extract meaningful information from high-dimensional omics datasets. By doing so, it creates a more holistic view of biological systems. Multi-omics approaches are becoming increasingly popular because they uncover relationships and interactions that single-omics strategies might overlook.
Historical significance
Historically, scientific research in biology has relied on isolated data sets. Genomics provided insights into genes and their functions, while transcriptomics focused on RNA levels. Proteomics looked at protein expressions, and metabolomics studied small molecules involved in metabolism. As technology advanced, the limitation of examining these data types in isolation became clear. Thus, the concept of multi-omics emerged, fundamentally changing how researchers approach biological questions.
Key Findings and Discussion
Major results of the study
The integration of multi-omics data has led to significant advancements in several areas of research. One notable finding is the ability to predict disease outcomes with greater accuracy. The synthesis of diverse data sets allows for a nuanced understanding of disease mechanisms, which can lead to improved therapeutic strategies.
Detailed analysis of findings
- Enhanced insights: By employing Mofa Multi Omics, researchers can identify biomarkers that have implications for both diagnosis and treatment.
- Challenges: Integrating massive datasets requires sophisticated computational methods and robust statistical analyses. This can be a barrier for some research teams.
- Applications in various fields: The implications of Mofa Multi Omics stretch across many domains including cancer research, personalized medicine, and environmental biology. Each application provides unique insights that can drive future studies forward.
In summary, the Mofa Multi Omics approach is set to revolutionize research methodologies across biological disciplines.
Researchers, educators, and the scientific community alike stand to benefit from the unfolding potential of this integrative framework. Understanding how these different omics layers interact contributes not only to academic knowledge but also to practical applications in health and disease management.
Preamble to Mofa Multi Omics
Mofa Multi Omics represents a significant approach in biological research, emphasizing the integration of various omics data to foster a deeper understanding of intricate biological systems. The importance of this methodology cannot be overstated, as it facilitates a holistic view of cellular processes and their interplay, which traditional single-omics studies may overlook. By combining layers of data from genomics, transcriptomics, proteomics, and metabolomics, researchers can achieve a more comprehensive analysis of biological phenomena.
Furthermore, Mofa Multi Omics enhances the potential for disease mechanism exploration and personalized medicine. Researchers can better identify biomarkers for diseases, leading to more effective diagnostics and treatments. Integrating diverse types of data allows for the detection of novel patterns and interactions that might remain hidden when studying each omics layer in isolation.
Considering the technological advances and growing data availability, Mofa Multi Omics opens up several avenues in biomedical research. Its adaptability means it draws interest from diverse fields, including systems biology, pharmacology, and bioinformatics. This article aims to dissect the fundamentals of Mofa Multi Omics and elaborate on its historical background, which underpins its current application and future potential.
Defining Mofa Multi Omics
Mofa Multi Omics refers to a methodological framework designed to amalgamate data from various omic disciplines. This approach ensures that valuable insights can derive from the synergy of different biological layers. The term 'Mofa' itself underscores a framework that is specifically structured for the integration, analysis, and interpretation of high-dimensional omics data. In essence, this framework allows the simultaneous analysis of multiple omic datasets, providing a mechanism that addresses complexity in biological interpretation.
This multifaceted approach acknowledges that biological systems do not function in silos. Rather, their interactions are dynamic and multifactorial. Therefore, an integrated assessment of genomic, transcriptomic, proteomic, and metabolomic data offers a complete narrative of cellular activities, significantly contributing to our overall understanding of organism behavior.
Historical Context and Development
The genesis of Mofa Multi Omics can be traced back to the evolutionary progression of omics studies themselves. Initially, research in genomics paved the way for the exploration of transcripts and proteins, with early efforts focusing on individual areas. Development in sequencing technologies has spurred the growth of transcriptomics and proteomics, thus setting a fertile ground for integrating these fields. As each branch of omics advanced, the inherent need for integration became more pronounced.
Pioneering studies highlighted limitations in single-omics methodologies, catalyzing efforts to create integrative frameworks. This collaborative spirit in research was evident in early 21st-century publications and conferences, fostering the convergence of different omics disciplines. Consequently, Mofa Multi Omics emerged as a robust methodology. By utilizing statistical models and data integration strategies, researchers can utilize the full spectrum of biological data, enabling more refined analyses than previously possible.
Fundamental Concepts of Omics
Omics science, which encompasses a range of biological fields, is crucial for understanding complex biological systems. It provides a framework to analyze biological data holistically, moving beyond the single-gene approach toward comprehensive insights into cellular processes. This article examines several omics layers that work together to enhance research in genetics, health, and disease.
Understanding these fundamental concepts is essential. It allows researchers to appreciate how individual omic layers interact and contribute to the larger biological picture. Recognizing these connections leads to more effective research strategies and improved interpretations of data.
Understanding Genomics
Genomics is the study of an organism’s complete set of DNA, including all of its genes. This field offers foundational knowledge that informs other omics disciplines. By sequencing genomes, scientists can identify genetic variations that may contribute to health conditions. Tools such as CRISPR-Cas9 have revolutionized genomics, allowing targeted edits in the genetic material. These advancements position genomics as a key player in fields like personalized medicine and biotechnology.
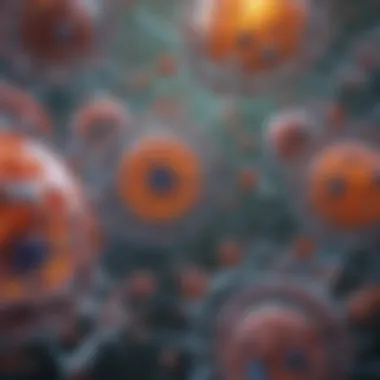
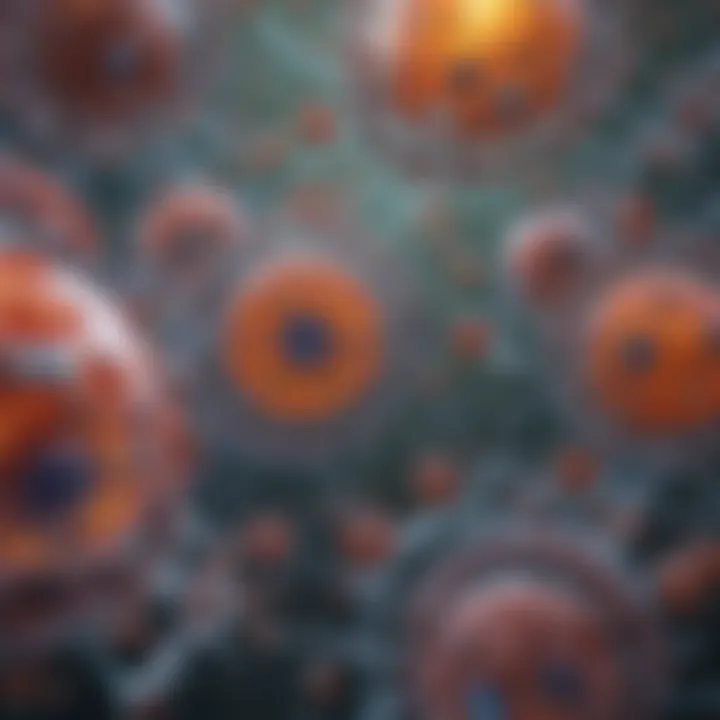
The data produced by genomic studies can guide researchers in identifying biomarkers for diseases, leading to more effective therapeutic strategies. Moreover, genomics contributes to evolutionary biology by providing insights into how species evolve over time.
Exploring Transcriptomics
Transcriptomics investigates the complete set of RNA transcripts produced by the genome under specific circumstances. It focuses on gene expression patterns, offering a dynamic view of cellular functions. Tracking RNA helps in understanding which genes are active at various times, influencing cell behavior and response.
Tools such as RNA-seq have enabled high-throughput analysis. Such methods have propelled the discovery of non-coding RNAs and alternative splicing events, which play critical roles in gene regulation. This knowledge is vital for developing therapeutic interventions in diseases like cancer, where gene expression alterations are common.
Insights into Proteomics
Proteomics is the large-scale study of proteins, particularly with respect to their functions and structures. Proteins are the products of gene expression and are therefore essential for understanding cellular mechanisms. Technologies like mass spectrometry allow comprehensive analysis of protein profiles, revealing how proteins interact in networks.
Studying proteomics enhances our understanding of cellular processes and disease mechanisms. For example, identifying specific protein alterations can indicate disease states, making proteomics invaluable for diagnostics and targeted therapies. Moreover, proteomic data can guide drug development, making it a key component of pharmaceutical research.
The Scope of Metabolomics
Metabolomics examines the complete set of metabolites in a biological sample. These small molecules are crucial indicators of cellular processes and reflect the state of the organism. Measuring metabolite levels can reveal how cells respond to environmental changes, genetic modifications, and disease states.
Metabolomics has wide applications in areas like toxicology and nutrition. Understanding metabolic profiles can lead to effective interventions in metabolic disorders. Additionally, it offers insights into pharmacokinetics and pharmacodynamics, assisting in drug development and personalized medicine approaches.
The Mofa Methodology Explained
The Mofa methodology plays a critical role in the landscape of multi-omics research. It offers a structured approach to aggregating and analyzing diverse biological data, ultimately contributing significantly to our understanding of complex biological systems. By employing rigorous methodologies, Mofa facilitates the integration of genomics, transcriptomics, proteomics, and metabolomics data. This integration is essential for elucidating the intricate interactions between different biological layers, such as how variations in gene expression might influence protein levels and metabolite production.
Mofa's approach not only brings forward a holistic view of biological activities but also addresses specific challenges such as data heterogeneity and dimensionality. The systematic strategies employed in Mofa serve to maximize data utility while minimizing the pitfalls often encountered in multi-omics analyses.
Data Collection Strategies
Data collection is the first pivotal step in the Mofa methodology. Accurate and efficient data gathering is vital to build a high-quality omics dataset. Several methods are utilized for this purpose, including:
- Sample Preparation: Choosing the right biological samples (e.g., tissues, cell cultures) ensures that the data is representative of the underlying biological processes.
- High-Throughput Technologies: Techniques such as next-generation sequencing for genomics and mass spectrometry for proteomics allow for comprehensive data collection, capturing a vast array of biological information in a relatively short amount of time.
- Standard Operating Protocols (SOPs): Adherence to standardized protocols reduces variation in data collection and promotes reproducibility.
These strategies emphasize the critical nature of initial data collection in shaping reliable analyses and interpretations.
Data Integration Techniques
The integration of diverse omics data is at the heart of the Mofa methodology. Data integration techniques developed in Mofa address the unique characteristics of each omics layer. Important techniques include:
- Multi-View Learning Techniques: These methods allow for the simultaneous analysis of multiple data types, thereby improving predictive accuracy and uncovering hidden biological patterns.
- Dimensionality Reduction: Techniques such as Principal Component Analysis (PCA) and Uniform Manifold Approximation and Projection (UMAP) help to condense large sets of data into more manageable forms without losing critical information. This helps researchers to visualize and interpret complex interactions more effectively.
- Statistical Fusion: Integrating data from various omics layers can be achieved using statistical models that account for correlation structures within the data. This approach supports drawing more robust conclusions from integrated datasets.
Effective data integration leads to discovering novel insights that may not be apparent within single-omics studies.
Statistical Models in Mofa
Statistical modeling in Mofa is crucial for interpreting the integrated omics data correctly. Several models are employed to analyze the data and make sense of underlying relationships:
- Bayesian Models: These models offer a flexible framework for incorporating prior knowledge into the analysis, thus enhancing the reliability of conclusions drawn from the data.
- Machine Learning Algorithms: Various machine learning techniques, such as clustering and regression models, are integral for unpacking the complex associations in multi-omics datasets.
- Hypothesis Testing Frameworks: Carefully designed statistical tests help determine if observed patterns in the data are significant or merely due to chance. This rigor is essential for validating research findings.
By employing these statistical models, Mofa enables researchers to extract actionable insights from multi-omics data, greatly contributing to the understanding of biological systems.
Applications of Mofa Multi Omics
The applications of Mofa Multi Omics represent a significant advancement in the field of biological research. This approach facilitates the integration of various omics data types to gain deeper insights into complex biological interactions. By analyzing diverse layers of information—such as genomic sequences, gene expression profiles, protein expressions, and metabolic pathways—researchers can explore multifaceted biological systems more thoroughly than with single-omic studies. The ability to combine these data types allows for a more comprehensive view of cellular mechanisms and disease progression, which is vital for advancing scientific knowledge and practical applications.
Disease Mechanism Exploration
Understanding the underlying mechanisms of diseases is a critical aspect of biomedical research. Mofa Multi Omics enables researchers to track molecular changes associated with disease processes. By integrating multiple omics layers, this methodology helps pinpoint specific biological markers that characterize particular diseases.
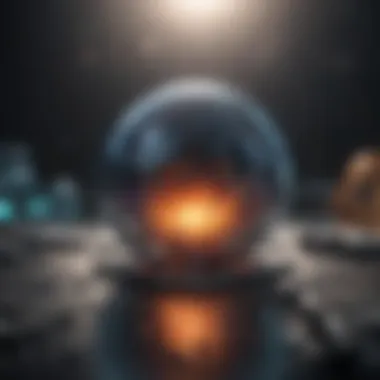
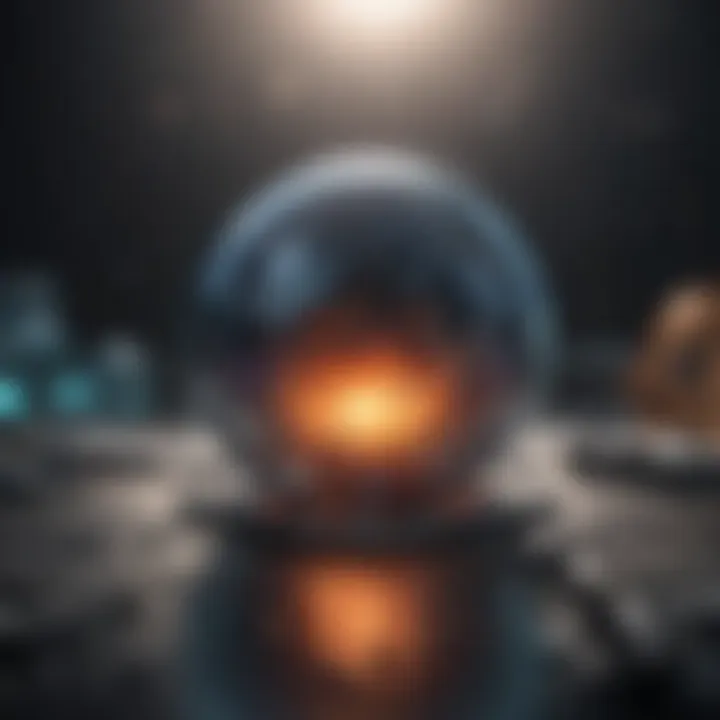
For instance, in oncological research, Mofa Multi Omics can reveal how variations in genomics translate into altered transcriptomics, subsequently affecting proteomics and metabolomics. This holistic view of cellular behavior can shed light on how cancers develop, progress, and respond to treatment. Moreover, it allows identification of potential therapeutic targets, ultimately informing strategies for precision medicine.
Key advantages of using Mofa Multi Omics in disease mechanism studies include:
- Identification of Biomarkers: Researchers can find unique biomarkers that indicate disease presence or progression.
- Understanding Pathways: It allows for the investigation of metabolic and signaling pathways affected by diseases, leading to the discovery of interconnections between different cellular processes.
- Personalized Approaches: By understanding how individual genetic and molecular profiles contribute to disease, clinicians can better tailor treatments to patient needs.
Drug Discovery and Development
The drug discovery process is traditionally time-consuming and expensive. However, Mofa Multi Omics can streamline this process significantly. By employing this integrated approach, pharmaceutical researchers can identify new drug candidates more efficiently and understand how these compounds will interact with various biological systems.
For example, Mofa Multi Omics can help researchers determine how specific proteins interact with drugs at the molecular level, assessing both efficacy and safety. It also assists in evaluating the metabolism of drugs within cells, predicting potential side effects and how these drugs may affect different pathways in varying patient populations.
The benefits of this application include:
- Increased Efficacy: By utilizing Mofa Multi Omics, researchers can identify compounds that are more likely to succeed in clinical trials based on comprehensive biological data.
- Reduced Failure Rates: Integrating omics data can help avoid costly late-stage failures by validating drug targets early in the pipeline.
- Innovative Therapies: The approach fosters the development of novel therapeutics by revealing unexplored biological mechanisms.
Overall, the applications of Mofa Multi Omics showcase a transformative potential to enhance understanding in diverse areas such as disease mechanisms, treatment options, and the innovative landscape of drug discovery.
Case Studies Illustrating Mofa Multi Omics
Case studies play a crucial role in understanding the real-world applications of Mofa Multi Omics. They showcase how integrating various omics data can lead to significant insights in biological research. By examining specific instances of Mofa's implementation, researchers can observe the effectiveness and versatility of the methodology. This section highlights the importance of these studies in bringing theoretical concepts into practical scenarios, thereby bridging the gap between laboratory findings and clinical applications.
Furthermore, case studies provide a reference point for future studies, outlining both successes and challenges faced during the application of Mofa Multi Omics. They serve as valuable resources for students and researchers alike, by highlighting specific elements such as:
- Approaches Taken: Different strategies used in applying Mofa across various research fields.
- Outcomes Achieved: Results and conclusions drawn from the integration of multiple omics layers.
- Challenges Encountered: Issues that arose during analysis or interpretation of data.
Oncological Research Applications
Oncology is one of the fields where Mofa Multi Omics has made notable contributions. The complexity of cancer requires a multifaceted approach for understanding its mechanisms. By integrating data from genomics, transcriptomics, proteomics, and metabolomics, researchers can obtain a more holistic view of tumor biology.
For instance, a study focusing on breast cancer utilized Mofa to analyze the interplay between genetic mutations and metabolic pathways. This allowed researchers to identify specific biomarkers that could predict treatment responses. Such insights could lead to more personalized treatment plans tailored to the individual characteristics of a patient’s tumor.
Moreover, employing Mofa in oncology aids in:
- Identifying New Targets: Discovery of novel therapeutic targets through multi-omics analysis.
- Understanding Heterogeneity: Deepening the understanding of tumor heterogeneity by profiling diverse omic layers.
- Predictive Modeling: Enhancing predictive models for patient outcomes based on integrated data.
Metabolic Disorder Insights
Mofa Multi Omics is also instrumental in exploring metabolic disorders. These disorders often involve complex interactions between various biological systems. Integrating omics data can uncover the biochemical pathways affected in conditions like diabetes, obesity, and metabolic syndrome.
In a notable study investigating type 2 diabetes, researchers applied Mofa to combine genomic and metabolomic data. This integration revealed previously undetected interactions between certain metabolites and genetic predispositions. Such findings not only enhance the understanding of diabetes pathophysiology but also open avenues for therapeutic innovations.
Key advantages of employing Mofa in metabolic disorder research include:
- Holistic Perspective: A comprehensive view of metabolic pathways and their regulation.
- Clinical Correlations: Establishing links between molecular alterations and clinical phenotypes.
- Potential for Intervention: Identifying biomarkers that could serve as targets for future interventions.
Integrating these diverse insights showcases the transformative potential of Mofa Multi Omics in the study of complex diseases. Through the lens of case studies, we can appreciate the practical applications and ongoing advancements in this evolving field.
Technical Challenges in Mofa Multi Omics
The exploration of Mofa Multi Omics presents significant technical challenges that researchers must address to fully harness its potential. These challenges stem from the inherent complexity of integrating diverse omics data types. Understanding these technical difficulties is crucial to advancing research in this field.
Data Quality and Standardization
Mofa Multi Omics relies heavily on data quality. Inconsistent data quality can lead to erroneous interpretations. Various data sources, such as genomics, transcriptomics, proteomics, and metabolomics, often have different standards and formats. Ensuring uniformity across these sources is essential. One major concern is missing or incomplete data. This can arise from poor experimental design or technical limitations in data acquisition. To mitigate this issue, researchers must employ robust data validation techniques.
Additionally, standardization in protocols is vital. Each omics field has its specific methods and techniques. For instance, differences in sample collection, preparation, and analysis can substantially affect outcomes. Therefore, creating standardized operating procedures can facilitate better data integration and collaboration among researchers. Tools and guidelines available on platforms like en.wikipedia.org help in achieving these standards.
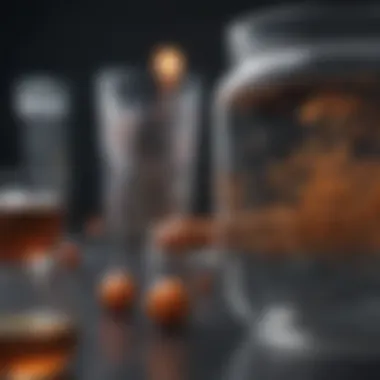
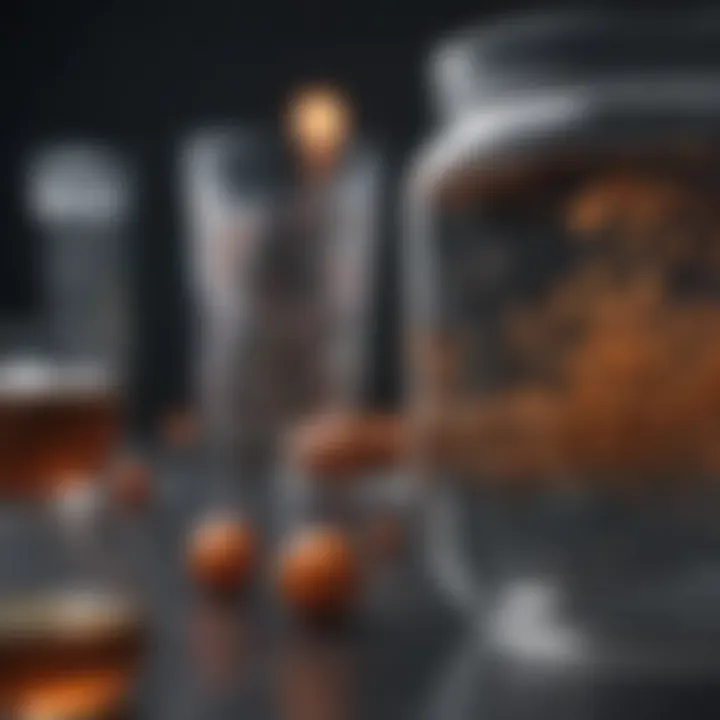
Data preprocessing is another critical aspect. This involves filtering out noise and relevant signals before analysis. Tools like R and Python offer packages for this purpose, allowing researchers to clean and normalize data sets.
"High-quality data ensures reliable results, leading to better insights in biological research."
Computational Complexity
Computational complexity represents another layer of challenge in Mofa Multi Omics. The integration of multiple omics layers generates vast amounts of data. Processing such data requires substantial computational power and sophisticated algorithms.
Algorithms used in Mofa must be capable of managing high-dimensional data. Moreover, feature selection becomes critical. Uninformative features may cloud meaningful insights, demanding strategies for their identification and exclusion. Researchers often utilize machine learning techniques to improve accuracy in predictions, although these methods require expertise in data science.
Moreover, as the complexity of data increases, so too does the demand for memory and storage. This can pose limitations on computational resources, especially for smaller institutions or individual laboratories. Collaborations and shared resources can alleviate some of these pressures, enabling access to high-performance computing.
Finally, interpretation of the integrated omics data can be non-linear and multifactorial. Researchers must navigate through layers of interactions and biological significance to derive meaningful conclusions.
Future Perspectives for Mofa Multi Omics
Future perspectives for Mofa Multi Omics signify an important junction in biological research and health sciences. This innovative approach harnesses the power of data integration from diverse omics layers. Such an integration paves the way for deeper insights. It moves beyond single omic layers to unveil complexities in biological systems.
The sophistication of biological phenomena requires advanced techniques. Mofa Multi Omics fulfills this need by processing and analyzing multifaceted data. The study of genomics, transcriptomics, proteomics, and metabolomics combined presents a holistic view. This makes it easier to comprehend disease mechanisms and therapeutic pathways. Researchers aim to elucidate how these different data types interact. Understanding these interactions has implications. It can lead to tailored therapies and interventions specific to individual patients.
Moreover, as the computational capabilities grow, so does the potential for integrating real-time data. This dynamism allows for continuous monitoring and adjustment of treatment strategies. Future advancements in this area may lead to a model of healthcare that shifts from reactive to proactive, improving patient outcomes.
Emerging Technologies
Emerging technologies play a crucial role in the future landscape of Mofa Multi Omics. The rise of artificial intelligence and machine learning is particularly noteworthy. These technologies can enhance data analysis, revealing patterns that may not be visible to human analysts. With improved algorithms, researchers can process large datasets more efficiently.
In addition, advances in high-throughput sequencing technology are rapidly changing the field. It allows for the generation of vast amounts of genomic data. Coupled with improved mass spectrometry for proteomics and metabolomics, researchers gain comprehensive insights into biological processes. Furthermore, innovations in data visualization tools aid in interpreting complex datasets, fostering better decision-making in research and clinical settings.
"Integrating Mofa Multi Omics with emerging technologies can potentially transform our understanding of biological functions and disease pathways."
Another promising development is the use of cloud computing. This technology provides scalable storage solutions and facilitates collaborative research efforts across borders. A unified platform can significantly enhance the access and sharing of omics data among researchers, which in turn can spur discoveries at a much faster pace.
Impact on Personalized Medicine
The impact of Mofa Multi Omics on personalized medicine is profound. As health professionals seek individualized treatment strategies, the importance of an integrated omics approach becomes evident. By understanding how various genetic, transcriptomic, proteomic, and metabolic factors interact in a patient, practitioners can devise more effective treatment plans.
The applications of Mofa in this regard are vast. Personalized medicine can address challenges in cancer treatment, where tumors are often genetically diverse. By using Mofa methodologies, oncologists can tailor therapies to the unique molecular profiles of individual tumors, leading to better patient responses and fewer side effects.
Another area is the management of chronic diseases. Conditions such as diabetes, cardiovascular disease, and neurological disorders involve complex interactions between genetic predispositions and environmental factors. Mofa Multi Omics enables a more comprehensive analysis of these interactions, leading to the prevention and early detection strategies that are highly individualized.
As healthcare moves towards a more tailored approach, the integration of various omics disciplines becomes even more critical. Researchers believe that as methods improve, Mofa Multi Omics could play a key role in the routine application of personalized medicine, unlocking new avenues for effective interventions.
Culmination: The Value of Integrating Omics Databases
The integration of omics databases is not just a technical achievement; it represents a paradigm shift in the way we understand biological systems. This section encapsulates the importance of Mofa Multi Omics and its role in advancing our knowledge of complex biological interactions. Mofa allows for a more nuanced view of cellular functions by combining various omic data types, leading to a more comprehensive analysis of biological phenomena.
There are several elements that underscore the value of integrating omics data. First, it enhances the analytical power needed to interpret multifaceted biological questions. By integrating genomics, transcriptomics, proteomics, and metabolomics, researchers can uncover patterns that may go unnoticed when analyzing each omic layer in isolation. Second, this integration fosters collaboration across disciplines. It engages researchers from various fields, enabling them to amalgamate different insights and methodologies, thus stimulating innovation.
Additionally, the combined insights from multi omics enable better predictive models. For instance, understanding how genetic variations affect metabolic processes can inform disease predictions and therapeutic strategies. This is particularly relevant in personalized medicine, where treatments can be tailored based on an individual’s unique biological profile.
Integrating omics databases leads to impactful discoveries and a more precise understanding of health and disease.
Overall, the value of integrating omics databases lies in its capacity to provide a holistic view of biology, fostering better research outcomes and practical applications in medicine and biotechnology.
Summarizing Key Findings
Mofa Multi Omics facilitates a comprehensive exploration into the dynamics of biological systems. The key findings can be summarized as follows:
- Data Synergy: The amalgamation of different omics layers provides richer insights into biological functions.
- Holistic Understanding: Each omic layer contributes a distinctive perspective, culminating in a fuller representation of biological processes.
- Predictive Analytics: Integrating data allows for improved models that can predict disease mechanisms and responses to therapies.
- Collaboration and Innovation: The methodology encourages interdisciplinary collaboration, enhancing the scope and breadth of scientific research.
Encouraging Future Research
Looking ahead, there is a clear need to encourage further research within the domain of Mofa Multi Omics. Several key areas present promising opportunities:
- Method Development: Advancements in computational tools and methods for better integrating omics data can drastically improve analytical accuracy.
- Standardization: Developing common standards for data collection and integration may enhance comparability across studies, fostering collaborative efforts.
- Application Expansion: There are vast, untapped opportunities to apply Mofa in new areas such as chronic disease management and environmental health, which require an integrated biological understanding.
- Education and Training: Educators must focus on equipping the next generation of scientists with skills in multi-omics methodologies to prepare them for future research challenges.