Exploring Computational Medicine and Bioinformatics
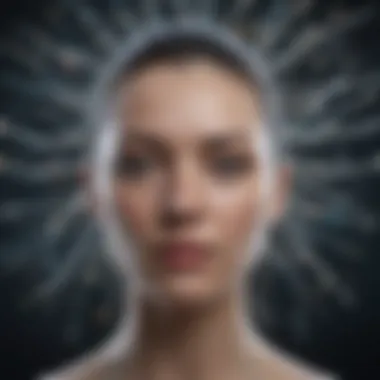
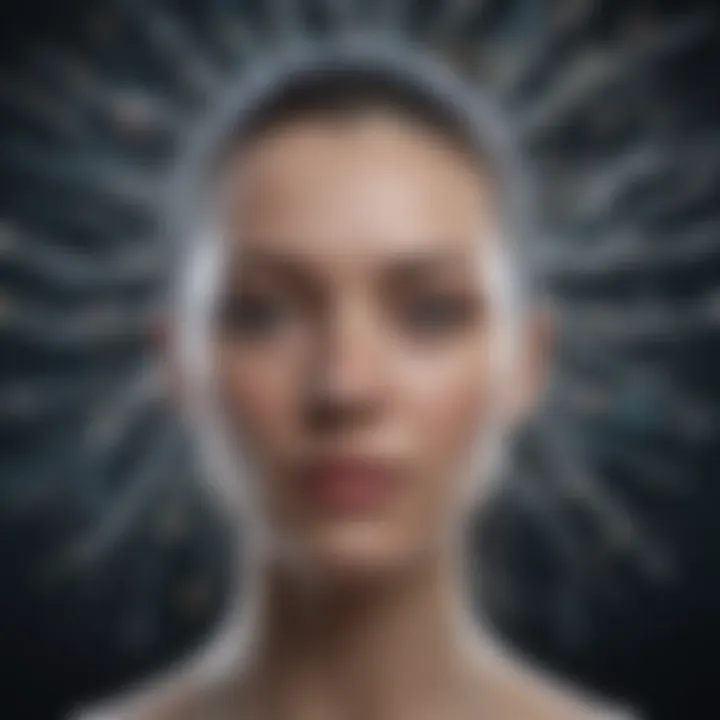
Intro
The integration of computational medicine and bioinformatics marks a significant shift in modern healthcare and research. It ushers in an era where technology is no longer a mere support system but rather an essential component in transforming patient outcomes and advancing scientific knowledge. This article seeks to explore these intertwined fields, unraveling their complexities while highlighting real-world applications and implications for the future of medicine.
Background and Context
Overview of the Research Topic
Computational medicine employs algorithms and analytical models to process vast amounts of health data. It goes beyond traditional practices by utilizing data from genomics, biomedical imaging, and electronic health records. On the other hand, bioinformatics focuses on applying computational techniques to manage biological data, particularly in genomics and proteomics. Together, these domains enable researchers and clinicians to derive insights that were once unattainable.
Historical Significance
Historically, advancements in healthcare have often been reliant on empirical observation. However, with the advent of computing technologies, a new paradigm emerged. The human genome project, completed in the late 1990s, served as a catalyst for the fields of bioinformatics and computational medicine. This monumental effort illustrated the impeccable potential of computational methods in interpreting genetic information. Over the years, various technological milestones such as machine learning, artificial intelligence, and big data analytics have further propelled these fields into mainstream medical applications.
Key Findings and Discussion
Major Results of the Study
Research indicates that integrating these fields significantly enhances disease diagnosis, treatment personalization, and predictive analytics. For instance, a study published in the journal Nature showcased how machine learning algorithms accurately predicted patient responses to specific cancer therapies, ultimately aiding oncologists in tailoring treatment plans.
Detailed Analysis of Findings
The application of algorithms in processing electronic health records has shown promise in identifying early warning signs of diseases. By analyzing patterns within these extensive datasets, healthcare professionals can intervene sooner, potentially improving patient outcomes.
Moreover, bioinformatics continually advances genomic research. By utilizing tools such as BLAST and genome browsers, researchers can interpret complex genetic data efficiently. This fosters innovations like targeted therapies, which have shown effectiveness in a range of diseases, notably in oncology.
Intro to Computational Medicine
Computational medicine represents a critical fusion of traditional medical practices and advanced computational techniques. In the contemporary healthcare landscape, this integration plays a vital role in enhancing patient care and research development. Through the application of data analytics, modeling, and algorithmic approaches, computational medicine addresses complex challenges in healthcare. Understanding this field is essential for students, researchers, and professionals alike, as it provides insights into how technology can optimize outcomes and improve the efficiency of medical practices.
Definition and Scope
Computational medicine can be defined as the interdisciplinary field that employs computational techniques to analyze biological and medical data. This encompasses a wide range of activities, including the analysis of clinical data, modeling of biological systems, and the use of algorithms to predict disease outcomes. The scope of computational medicine extends from bioinformatics to personalized medicine, offering tools and methods that enhance clinical decision-making and facilitate innovative research.
Historical Context
The origins of computational medicine are rooted in the growth of both medicine and computer science. The significant advancements in computing technology during the late 20th century laid the foundation for its emergence. Initially, medical professionals utilized simple computing tools to manage patient data. Over time, the field evolved with the development of sophisticated algorithms and software capable of analyzing complex biological datasets. Landmark projects like the Human Genome Project highlighted the potential of computational methods, enabling researchers to gather, store, and analyze vast amounts of genetic information. This historical progression illustrates how computational medicine has transitioned from basic data management to a cornerstone of modern healthcare.
Fundamental Principles
The fundamental principles of computational medicine revolve around several key concepts:
- Data Integration: Combining diverse datasets from various sources is essential for comprehensive analysis. This includes clinical records, genomic data, and environmental factors.
- Modeling and Simulation: Using mathematical models to simulate biological processes allows researchers to predict outcomes and test hypotheses in a virtual environment.
- Algorithm Development: Creating and refining algorithms that can make sense of complex data patterns boosts the predictive accuracy of diagnostics and treatment plans.
- Collaboration: Interdisciplinary collaboration is crucial. Healthcare professionals, data scientists, and bioinformaticians must work together to leverage strengths from each discipline.
"Computational medicine provides new pathways for understanding diseases, tailoring treatments to individual patients, and improving overall healthcare outcomes."
Overall, the principles behind computational medicine guide its application in real-world scenarios, helping to elevate its impact on both healthcare delivery and research.
Bioinformatics Overview
Bioinformatics is a crucial aspect of the intertwined fields of computational medicine and bioinformatics. It acts as a bridge that enhances our understanding of biological data. The integration of biology, computer science, and information technology provides a framework for analyzing complex biological data. This overview serves to highlight the significance of bioinformatics in modern medicine and research.
Definition and Importance
Bioinformatics can be defined as the application of computational tools and techniques to manage, analyze, and interpret biological data. In today's world, where large datasets generate rapidly, bioinformatics has become indispensable. It is important due to several reasons:
- Data Management: Bioinformatics deals with large-scale biological data, facilitating storage and retrieval.
- Genomic Research: It supports genome sequencing, allowing researchers to understand genetic variations.
- Systems Biology: Integrates information from various biological systems, providing holistic views.
The analytical capabilities of bioinformatics streamline the process of translating complex biological questions into actionable insights.
Evolution of the Field
The field of bioinformatics has witnessed significant evolution, beginning in the 1960s. Early efforts revolved around sequence alignment tools and databases. The development of genomic technologies further accelerated growth. Key milestones include:
- The mapping of the human genome served as a catalyst for bioinformatics applications.
- Emergence of software like BLAST (Basic Local Alignment Search Tool) paved the way for sequence searches.
- The rise of machine learning approaches has transformed data analysis efficiency.
This evolution highlights how bioinformatics continuously adapts to new technological advancements to meet scientific demands.
Core Technologies in Bioinformatics
Several core technologies underpin bioinformatics, enabling it to function effectively. These technologies include:
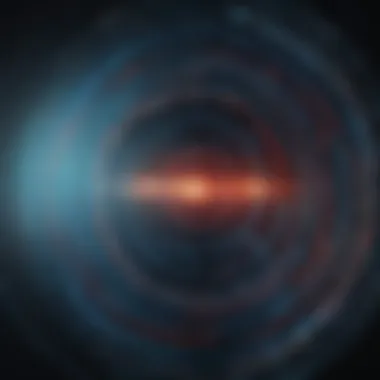
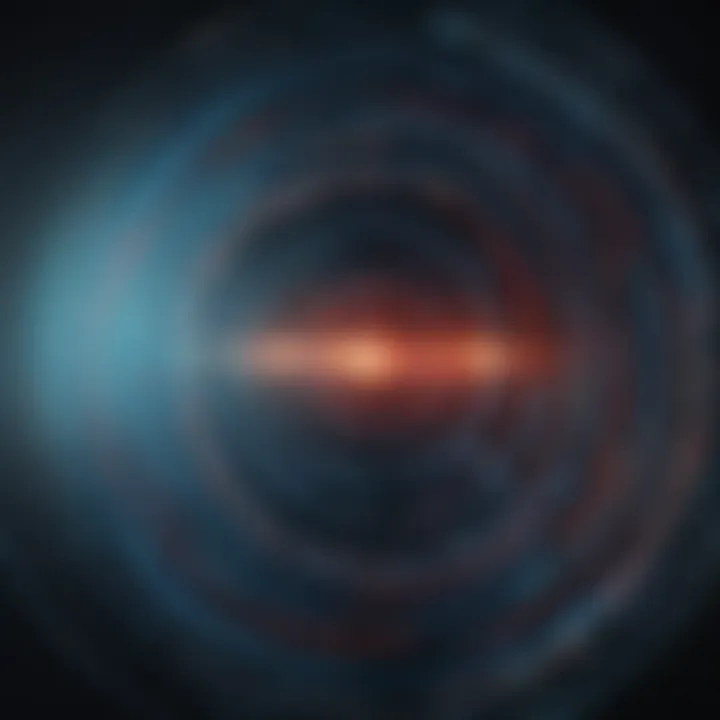
- Databases: Repositories like GenBank store genetic sequences, providing easy access for researchers.
- Algorithms: Computational algorithms, such as those for sequence alignment, are essential for data analysis.
- Machine Learning: With its ability to analyze patterns in large datasets, machine learning enhances predictive capabilities.
- Bioinformatics Tools: Software such as Galaxy and Bioconductor provide platforms for data analysis and visualization.
These technologies form the backbone of bioinformatics, empowering scientists and researchers to derive meaningful conclusions from complex datasets.
Bioinformatics is not just about managing data; it creates opportunities for breakthroughs in biomarker discovery and personalized medicine, impacting healthcare profoundly.
Relationship Between Computational Medicine and Bioinformatics
The relationship between computational medicine and bioinformatics is fundamental to the evolution of modern healthcare. Understanding this relationship reveals how the integration of these disciplines can improve both patient outcomes and medical research. The multidisciplinary approach has highlighted the importance of data analysis, algorithm development, and biological insights all working together.
Interdisciplinary Nature
Computational medicine and bioinformatics are rooted in diverse disciplines. Computational medicine often draws from fields like computer science, engineering, biology, and medicine. Similarly, bioinformatics relies on mathematics, statistics, and various biological sciences. This cross-disciplinary approach fosters innovation. It allows researchers to analyze health data more effectively. For example, bioinformatics provides the tools to interpret complex biological data, which computational medicine then uses to develop predictive models for disease progression.
Collaboration between biologists and data scientists is crucial. Without the synergy of expertise, optimal outcomes could not be achieved. Health professionals can leverage the intersections of these fields to create tailored treatment plans, while researchers can focus on uncovering unseen patterns in large datasets.
Complementary Roles in Research
The complementary roles these disciplines play in research cannot be overstated. Bioinformatics contributes to data management, allowing vast biological datasets from genomics and proteomics to be analyzed efficiently. This is particularly useful when interpreting high-throughput sequencing results. In turn, computational medicine utilizes these analyses to inform clinical decisions.
An example is in cancer research, where bioinformatics tools analyze genetic mutations linked to various cancers. Computational models can then simulate the effects of these mutations on treatment outcomes. By integrating this information, researchers can develop more effective personalized therapies. Hence, the relationship enhances both the understanding of disease mechanisms and the efficacy of interventions.
Case Studies of Cross-Applications
Real-world examples underscore the effectiveness of combining computational medicine and bioinformatics. One prominent case is the use of genomic information in oncology. With the advent of Next-Generation Sequencing technologies, patient tumor samples can be sequenced to identify specific mutations. Bioinformatics platforms aid in managing and interpreting this data. The use of computational models allows oncologists to predict which therapies may be most effective based on the tumor's genetic profile.
Another case study involves infectious diseases. During the COVID-19 pandemic, bioinformatics tools were essential for tracking virus mutations and spread patterns. Computational models were used to forecast outbreaks, informing public health responses. This integration of disciplines provided an essential framework for managing the crisis effectively.
"Understanding the relationship between computational medicine and bioinformatics is imperative for driving future innovations in healthcare."
These case studies highlight how overlapping knowledge and techniques can shape advancements in medical science. Understanding these connections opens avenues for potential breakthroughs, ultimately enhancing healthcare effectiveness and research.
Computational Tools and Methods
The integration of computational tools and methods is critical in the study of medical and biological data. They serve as the backbone for analyzing vast quantities of information generated in healthcare and research settings. As the fields of computational medicine and bioinformatics become more interconnected, the significance of these tools increases. They enable researchers and practitioners to derive meaningful insights from complex datasets, thereby enhancing decision-making processes in medical settings.
Machine Learning Algorithms
Machine learning algorithms are at the forefront of innovation in healthcare. They allow for the analysis of large datasets to discover patterns that are not immediately evident. The ability to learn from data without explicit programming makes these algorithms particularly useful in identifying disease trends and predicting patient outcomes.
In computational medicine, algorithms like decision trees, support vector machines, and neural networks find wide application. For example, a neural network may analyze imaging data from MRI scans to assist in diagnosing tumors. The algorithms learn from previous data, refining their accuracy as they process more information.
Machine learning not only enhances diagnostics but also personalizes treatment plans, providing the right care to the right patients.
Data Mining Techniques
Data mining techniques facilitate the extraction of useful information from large datasets. These approaches help in recognizing patterns and correlating features that can lead to better patient management and effective healthcare strategies. Unsupervised learning, clustering, and association rule learning are often used in bioinformatics to find relationships among biological data.
For instance, clustering techniques can categorize patients based on genetic information, leading to more targeted therapies. Additionally, association rule learning can uncover links between drug interactions and patient responses. As such, data mining plays a vital role in advancing personalized medicine and crafting effective intervention strategies.
Statistical Approaches
Statistical approaches are foundational for drawing valid conclusions from data. These methodologies support hypothesis testing, estimation, and predictive modeling. In computational medicine, they help validate findings and ensure that results are statistically significant. Common techniques include regression analysis, hypothesis testing, and survival analysis.
In drug discovery, for example, statistical approaches are employed to assess the effectiveness of new medications against existing treatments. By analyzing trial data for disparities in performance, researchers can make informed decisions about drug efficacy. The importance of statistics in understanding variability in treatment outcomes cannot be overstated.
In summary, computational tools and methods encompass a range of techniques essential for both computational medicine and bioinformatics. Understanding and utilizing these tools is crucial in addressing contemporary health challenges.
Applications in Healthcare
The integration of computational medicine and bioinformatics is reshaping the healthcare landscape significantly. Applications in this domain are critical, as they address specific healthcare challenges and enhance patient outcomes through targeted interventions. By leveraging algorithms, big data, and genomics, practitioners can now offer personalized care that was previously unattainable.
Personalized Medicine
Personalized medicine emphasizes tailoring medical treatment to the individual characteristics of each patient. This approach relies heavily on genomic data, enabling healthcare providers to make informed decisions based on unique genetic profiles. By analyzing these profiles, clinicians can identify the most effective treatment strategies, minimizing trial and error in prescribing medications.
Moreover, personalized medicine incorporates patient lifestyle and environmental factors into treatment plans. For instance, certain drugs may be more effective in specific populations, and genomic research can reveal these tendencies. As a result, patients experience fewer side effects and more effective treatments. In summary, personalized medicine marks a pivotal shift in healthcare, harnessing the power of computational techniques to optimize individual care.
Predictive Analytics in Disease Prevention
Predictive analytics involves the use of statistical algorithms and machine learning techniques to identify potential health risks before they manifest. This proactive approach is especially valuable in public health, where early detection can lead to timely interventions. For example, algorithms can analyze data from various sources, such as electronic health records and social media, to predict outbreaks of infectious diseases.
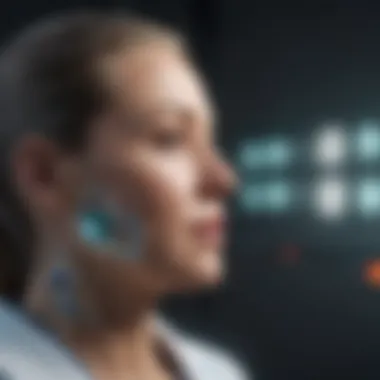
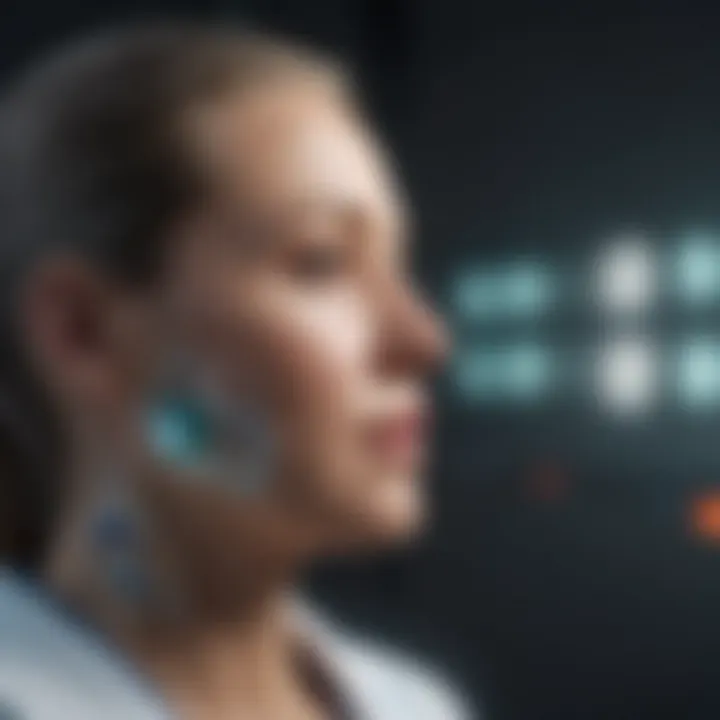
The potential of predictive analytics extends beyond infectious diseases; it also applies to chronic conditions. By integrating personal medical history and lifestyle choices, healthcare providers can forecast the likelihood of conditions like diabetes or cardiovascular diseases. This data-driven methodology empowers patients to adopt preventative measures early on.
"Predictive analytics not only enhance individual outcomes but also optimize resource allocation in healthcare systems."
Drug Discovery and Development
The process of drug discovery and development is extremely complex, often taking years and substantial financial investment. However, computational medicine combined with bioinformatics is transforming this area, making it more efficient and less costly. The ability to process vast amounts of biological data allows researchers to identify potential drug targets faster.
Techniques such as molecular docking simulations can predict how drugs interact with specific proteins at the molecular level. This leads to the identification of promising compounds that can be further developed into therapeutic agents. Moreover, data-driven approaches allow for the analysis of clinical trial outcomes, improving the selection of patient populations for trials and increasing the likelihood of drug approval.
Ethical Considerations
The role of ethical considerations in computational medicine and bioinformatics cannot be overstated. As these fields evolve, they encounter complex situations that require careful handling of sensitive data, fairness in model development, and adherence to regulations. Understanding these ethical dimensions is crucial not just for researchers, but for the integrity of the healthcare system as a whole.
Data Privacy Concerns
One of the paramount issues in computational medicine and bioinformatics is data privacy. Patient data is often sensitive and requires fundamental protections to prevent misuse. The Health Insurance Portability and Accountability Act (HIPAA) in the United States provides a framework for safeguarding this information. However, with advancements in data analytics and machine learning, the risk of breaches increases. Continuous efforts are needed to ensure robust encryption methods and access controls.
Moreover, engaging patients in discussions regarding how their data is being used fosters trust and ensures informed consent. Researchers and healthcare professionals must prioritize transparent communication regarding data-sharing practices. The integration of anonymization techniques can help mitigate risks but must be carefully balanced against the need for data utility in research.
Bias in Computational Models
The presence of bias in computational models stands as a significant ethical concern. If the data sets used to train predictive algorithms are not representative of the population, the outcomes can be skewed, leading to unequal treatment for specific groups. For instance, algorithms derived from homogeneous datasets may not account for diverse genetic backgrounds or social contexts, thus perpetuating health disparities.
To counteract this, practitioners must implement rigorous validation processes that test the models across various demographics. It's vital to consciously curate training data to reflect the diversity of the patient population. Engaging a diverse team in the development process can also bring multiple perspectives that help identify and rectify potential biases before operational deployment.
Regulatory Challenges
Navigating the regulatory landscape presents another complex layer in the ethical discourse surrounding computational medicine and bioinformatics. Regulatory bodies often lag behind technological advancements, making it difficult to establish consistent guidelines. This inconsistency can lead to fragmentation in compliance and risks to patient safety.
To tackle these challenges, collaboration between technologists, ethicists, and regulators is essential. Developing contemporary frameworks that can adjust to rapid innovations is critical for the protection of patient rights and data integrity. The need for ongoing education about regulatory requirements also cannot be neglected, as it ensures all stakeholders understand their responsibilities.
Ethical considerations in computational medicine and bioinformatics are not merely an afterthought; they are foundational to enhancing healthcare outcomes and building public trust.
Future Trends in Computational Medicine and Bioinformatics
The intersection of computational medicine and bioinformatics is rapidly evolving, with new trends that promise to redefine healthcare practices. Understanding these trends is vital for students, researchers, and professionals in the field. Keeping abreast of future developments will enhance the ability to leverage computational techniques for better patient outcomes.
Integration of Artificial Intelligence
Artificial Intelligence (AI) is at the forefront of transforming computational medicine and bioinformatics. By applying machine learning and deep learning techniques, AI can analyze vast datasets efficiently. This capability enables the identification of patterns, which can lead to more accurate predictions of disease progression and responses to treatment.
Key benefits of AI integration include:
- Enhanced Diagnostic Accuracy: AI algorithms can support clinicians by providing second opinions and highlighting unusual patterns in medical imaging or genomic data.
- Personalized Treatment Plans: AI can help in tailoring specific interventions based on an individualโs unique genetic makeup.
However, there are also considerations that need attention. One significant challenge is the need for high-quality training data. If the data used for training models contains biases, the outputs can lead to erroneous conclusions in clinical settings. Thus, continuous scrutiny and refinement of data sources are essential for the advancement of AI applications in this domain.
Advancements in Genomics
The field of genomics is making remarkable strides, which in turn influences computational medicine. With the cost of sequencing continuing to fall, genomic data is becoming more accessible. Advances in technologies such as CRISPR and next-generation sequencing are providing researchers with better tools to understand genetic diseases.
Highlighted advancements include:
- Whole Genome Sequencing: Researchers can now sequence entire genomes quickly and affordably, unlocking vast amounts of information about genetic variations.
- Gene Editing Technologies: Innovations in gene editing allow for targeted therapies, providing the potential for correcting genetic defects.
As these technologies advance, the integration of genomic data into clinical workflows will become more prominent. It is crucial to ensure that clinicians are adequately trained to interpret genomic data, linking it to therapeutic decisions.
Emerging Technologies
In addition to AI and genomics, several emerging technologies are reshaping the landscape of computational medicine and bioinformatics. These technologies offer new opportunities for research and healthcare improvement.
Some noteworthy emerging technologies include:
- Wearable Devices: These devices can continuously monitor patient health metrics, generating extensive datasets that can inform patient management and preventive care strategies.
- Blockchain for Data Security: This technology provides a secure framework for storing sensitive medical information, ensuring privacy and compliance with regulations.
- Cloud Computing: The cloud allows for greater collaboration across research institutions, fostering an environment for sharing data and resources effectively.
As these technologies develop, they bring both potential benefits and challenges. Ensuring data integrity and adherence to ethical standards will be paramount in harnessing the power of these innovations in healthcare.
"The future of computational medicine and bioinformatics lies in our ability to integrate technology more seamlessly into healthcare practices, ensuring both predictive capabilities and ethical integrity."
In summary, the future trends in computational medicine and bioinformatics highlight the potential for improved patient care through advanced technologies. Keeping pace with these trends is not only crucial for professionals in the field, but it is also essential for fostering an environment of innovative research that prioritizes patient well-being.
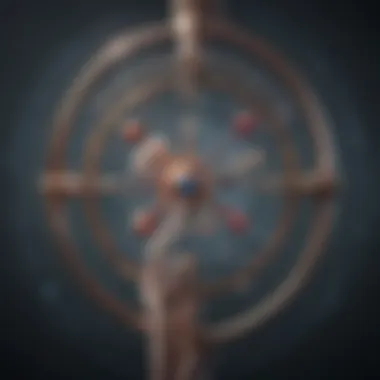
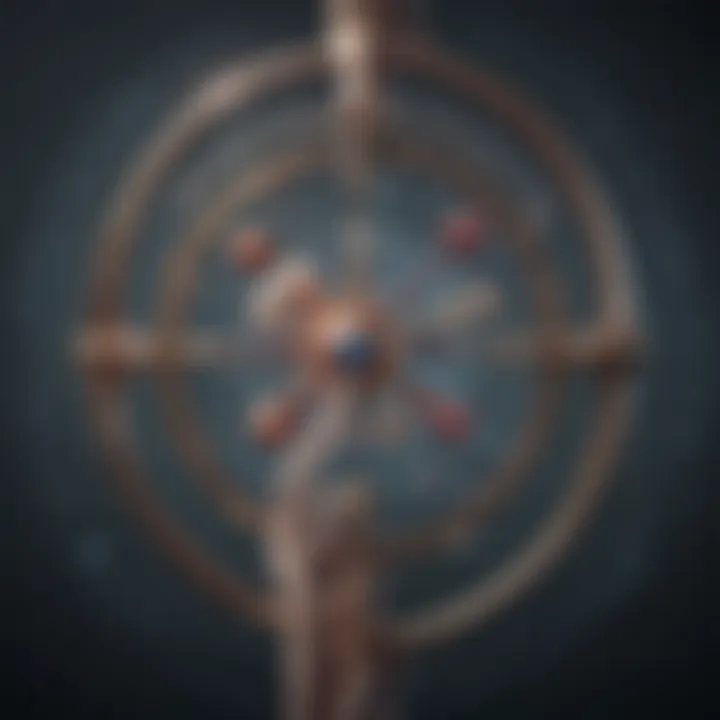
Challenges and Barriers
The synthesis of computational medicine and bioinformatics is not without its challenges. Understanding these barriers is essential for anyone involved in these fields. Addressing constraints can lead to significant advancements and improvement in healthcare outcomes. Discussion of such challenges fosters awareness among students, researchers, and professionals, guiding them to innovate effectively in these rapidly evolving domains.
Technical Limitations
Technical limitations represent a significant barrier in the integration of computational medicine and bioinformatics. Systems often face issues related to data quality, computational power, and software complexity. Computational models require vast amounts of high-quality data to function accurately. However, data may be noisy, incomplete, or inconsistent. The challenge lies in ensuring that the data used is both comprehensive and precise.
Moreover, the algorithms employed in computational models may not always generalize well to different datasets. This can lead to biases or inaccurate predictions in real-world applications. With the increasing amount of biological data generated, the ability to process and analyze this information efficiently is vital. High-performance computing resources can help tackle some of these issues but are not universally accessible.
Funding and Resources
Another significant barrier within computational medicine and bioinformatics is the scarcity of funding and resources. Research and development in these fields require substantial financial investment. This funding is often allocated based on preliminary results or institutional priorities, causing fluctuations in research continuity. Consequently, smaller research groups or independent researchers may struggle to secure the necessary funding to advance their work.
Additionally, the competition for grants can lead to underfunded areas within computational medicine and bioinformatics. Limited resources can restrict access to cutting-edge technology and tools essential for innovation. As a result, researchers might not explore new approaches or technologies, hindering the entire field.
Interdisciplinary Collaboration
Interdisciplinary collaboration poses challenges but is critical for driving advancements. Effective cooperation between experts in computational medicine, bioinformatics, biology, and clinical settings requires a shared understanding of diverse scientific languages and methodologies. Miscommunication or lack of appreciation for different perspectives can create hurdles.
Additionally, team dynamics often suffer if roles and responsibilities are not clearly defined. It is essential to establish a culture of collaboration that encourages mutual respect and cohesion among interdisciplinary teams. When specialists work together, they can leverage their expertise to address complex health issues, leading to innovative solutions. Hence, fostering a collaborative environment is pivotal for overcoming these challenges and fostering the growth of computational medicine and bioinformatics.
Case Studies and Success Stories
The discussion of case studies and success stories in the fields of computational medicine and bioinformatics serves as a critical avenue for understanding the real-world impacts of these disciplines. By analyzing tangible examples, researchers and practitioners can better grasp how theoretical frameworks translate into practical applications. This section highlights significant breakthroughs that exemplify the power of these interlinked fields, indicating their relevance for ongoing and future health initiatives.
Innovations in Cancer Research
Cancer research has greatly benefited from computational medicine and bioinformatics. In recent years, initiatives like The Cancer Genome Atlas have utilized vast data sets to provide insights into tumor heterogeneity and patient stratification. Researchers apply bioinformatics tools to analyze genomic data, revealing distinct subtypes of cancer. Such analyses often guide personalized treatment plans, optimizing therapeutic responses for individual patients.
Furthermore, machine learning algorithms play a crucial role in identifying patterns within patient data, which can lead to early detection of malignancies. For example, software that employs artificial intelligence has been developed to evaluate medical images and thereby improve diagnostic accuracy. Recent studies have shown promising results in using these algorithms for predicting outcomes and identifying potential targets for therapies.
Neuroscience Breakthroughs
In neuroscience, the intersection of computational medicine and bioinformatics is reshaping our understanding of complex neurological disorders. The analysis of neural data through advanced computational tools has led to groundbreaking discoveries regarding conditions like Alzheimer's disease and multiple sclerosis. By employing statistical modeling techniques, researchers can map the progression of these diseases in a more detailed manner than previously possible.
Additionally, neural network models are being used to analyze brain imaging data to identify biomarkers associated with various neurological conditions. This kind of analysis can improve diagnosis and facilitate targeted interventions, thus enhancing patient outcomes significantly. One noteworthy example is the use of brain-computer interfaces, which leverage bioinformatics approaches to aid patients with movement impairments.
Infectious Disease Modelling
Infectious disease modelling is another crucial area where computational medicine and bioinformatics have shown notable success. During the COVID-19 pandemic, bioinformatics tools were essential in tracking variants of the virus. Researchers utilized genomic sequencing data to monitor mutations and predict their potential impact on transmissibility and vaccine effectiveness. Collaborative platforms, such as GISAID, have shown the importace of shared data in combatting global health threats.
Moreover, computational models have been employed to understand transmission dynamics, effectively informing public health policy and intervention strategies. These models allow health authorities to evaluate potential outcomes of various strategies, providing crucial insights into reducing the spread of diseases.
"The integration of computational techniques with traditional biomedical practices has the potential to accelerate innovation in health sciences dramatically."
Through these case studies, it is clear that computational medicine and bioinformatics not only advance scientific knowledge but also have real implications for patient care and public health. The continual evolution of these fields promises further insights and breakthroughs, ensuring their importance in future healthcare advancements.
Educational Resources and Training Programs
Educational resources and training programs play an essential role in bridging the gap between computational medicine and bioinformatics. The integration of advanced computation and biological data analysis into healthcare demands a skilled workforce that understands both fields. A robust educational framework fosters innovation and ensures that professionals are well-equipped to navigate the complexities that arise within these intersecting domains. Key benefits include enhanced interdisciplinary collaboration, improved research methodologies, and ultimately, advanced patient care.
Formal Education Pathways
Formal education acts as the foundation for expertise in computational medicine and bioinformatics. Universities and colleges now offer specialized degree programs that combine elements from both disciplines. These programs typically cover subjects such as computer science, data analysis, biostatistics, and molecular biology. Students learn to apply computational techniques to solve biological problems, paving the way for roles in research and healthcare. Institutions like Stanford University and Johns Hopkins University provide excellent examples of comprehensive curricula that encourage this interdisciplinary approach. Through structured courses and hands-on projects, graduates emerge with competencies that are vital for success in modern healthcare environments.
Online Learning Platforms
Online learning platforms are increasingly popular for professionals seeking to update or enhance their skills without the commitment of a full degree program. Websites such as Coursera, edX, and Udacity offer courses focused on data science, bioinformatics, and machine learning. Many courses are designed by leading universities and industry experts, which provides credibility and insight into current trends. For instance, courses on genomic data analysis or predictive modeling can be invaluable for those in the field. These platforms allow for flexibility, enabling learners to explore complex topics at their own pace, often leading to a rich understanding of both computational and biological aspects.
Interdisciplinary Workshops
Interdisciplinary workshops serve as collaborative spaces where professionals from various backgrounds come together to exchange knowledge and ideas. These workshops often attract students, researchers, and industry experts, creating a vibrant environment for innovation. For example, events like the bioinformatics hackathons or computational biology symposiums encourage teamwork to tackle real-world challenges. Participants gain practical experience on current technologies and methodologies, which can be applied within their own work environments. Such engagements not only enhance learning but also foster networking opportunities that may lead to future collaborations.
"Continual learning and adaptation are key in the rapidly evolving fields of computational medicine and bioinformatics."
Epilogue
The intersection of computational medicine and bioinformatics signifies a crucial advancement in our understanding of health and disease. This integration paves the way for innovative healthcare solutions, enhancing patient care through informed decision-making and tailored treatments. The topics covered in this article illustrate the transformative potential of combining computational techniques with biological insights.
Summary of Key Insights
This section summarizes the core ideas derived from our exploration of computational medicine and bioinformatics:
- Interdisciplinary Collaboration: Integration between diverse fields such as computer science, biology, and medicine encourages diverse perspectives to solve complex healthcare problems.
- Technological Advancements: Machine learning, data mining, and statistical approaches are fundamental in reshaping our approach to health challenges, offering precise and actionable insights.
- Practical Applications: From personalized medicine to drug discovery, applying computational methods directly impacts treatment paths and patient outcomes.
- Ethical Implications: With advancing technologies, ethical considerations, like data privacy and biases, must be thoughtfully considered to ensure equitable healthcare for all.
- Future Prospects: Ongoing advancements in genomics and artificial intelligence hint at a promising future, where healthcare can continuously evolve to meet the needs of patients effectively.
The continued evolution of computational medicine and bioinformatics is pivotal for realizing a sophisticated and integrated healthcare system, one that prioritizes patient outcomes and quality of care.
Understanding these aspects not only benefits healthcare professionals but also empowers patients to engage in their health decisions more actively. Thus, this conclusion serves as a reminder of the vital role these disciplines play in shaping the future of healthcare.