Artificial Technology: Innovations and Implications
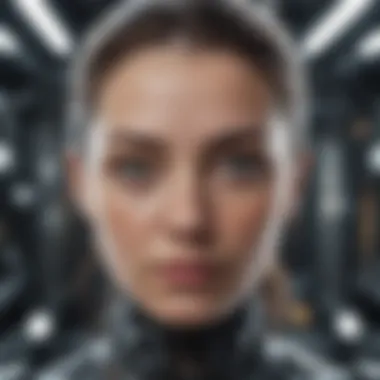
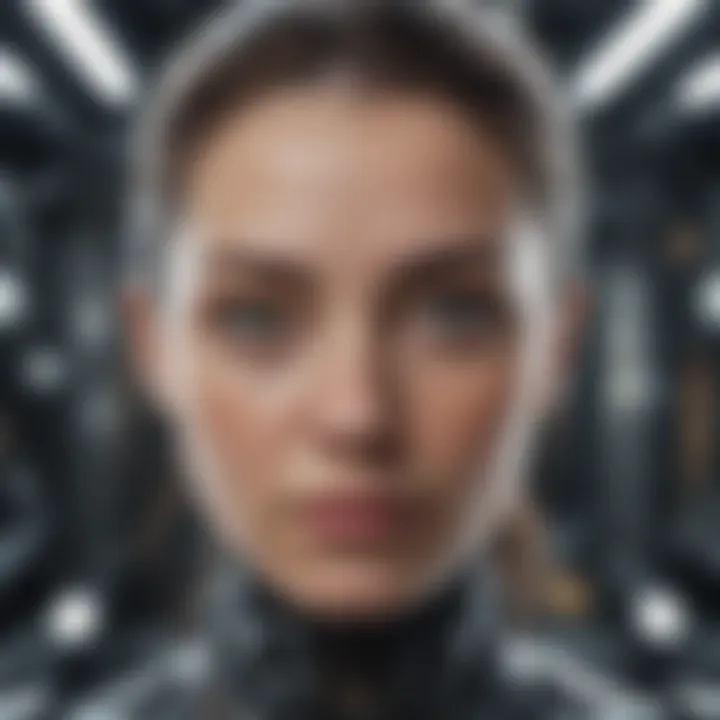
Background and Context
Artificial technology, often interchangeably referred to as artificial intelligence or AI, has solidified itself as a dominant force impacting numerous facets of society. This section attempts to lay a foundation for understanding the nuances of this expansive field, which has transformed from a theoretical concept into a vital component of modern technology.
Overview of the Research Topic
The evolution of artificial technology illustrates its ambitious journey from the early days of theoretical discussions to developments that now inform our daily lives. Notably, the integration of machine learning algorithms, natural language processing, and robotic process automation serve as pillars supporting this evolution. As technology enthusiasts and professionals delve deeper, it becomes clear that the interaction between humans and machines is no longer just reactive; rather, it is proactive, creating an environment where machines learn and adapt in real-time.
Historical Significance
To appreciate where artificial technology is headed, one must glance backwards. The genesis can be traced to the 1950s, with pioneers like Alan Turing and John McCarthy laying the groundwork. Turingâs seminal question, "Can machines think?" sparked debates that are still relevant today. McCarthyâs invention of the term "artificial intelligence" marked a significant moment, connoting not just the mechanization of tasks but the prospect of machines simulating human thought.
As decades unfolded, pioneering moments emerged, such as the development of expert systems in the 1970s and the AI winter of the late 1980s that followed a period of diminished funding and enthusiasm. It wasnât until the turn of the millennium, with the rise of big data and enhanced computational power, that the landscape transformed like never before.
"Artificial intelligence represents the ultimate tool; its ability to learn can spark both miraculous advancements and troublesome dilemmas in equal measures."
Key Findings and Discussion
Major Results of the Study
Research into artificial technology reveals sheer potential alongside stark challenges it faces. From all corners, industries such as healthcare, finance, and entertainment are adopting these innovations, showcasing remarkable improvements in efficiency and user engagement. In healthcare, for instance, AI-powered diagnostics have outperformed traditional methods in both speed and accuracy, highlighting a shift in patient care.
Detailed Analysis of Findings
However, this rapid advance invites critical scrutiny regarding ethical implications. For example, how do we address biases inherent in machine learning models which can lead to unfair treatment in hiring processes? The conversation about ethics is not merely about questions of right and wrong; rather, it dives into the core of what it means to trust a system that continues to learn independently.
Comparing regions also illuminates stark disparities. In some parts of the world, access to artificial technology is a privilege of a few, while in others, it's an essential utility catering to various needs. Understanding this global landscape help frame discussions about equity, access, and the future of work.
In closing, the exploration of artificial technology promises to deepen, intertwining various disciplinary threads and showcasing how its innovations can either bridge gaps or widen them, depending on the societal context in which they are deployed. The implications, both positive and negative, warrant continued scrutiny and dialogue.
Prelims to Artificial Technology
Artificial technology isnât just a buzzword; itâs a transformative force that has woven itself into the very fabric of modern life. Understanding the foundations and implications of this technology is crucial for anyone looking to navigate todayâs rapidly evolving landscape. As we step deeper into the digital age, the influence of artificial intelligence (AI) permeates almost every sector we can fathom, altering the way we work, communicate, and live.
Defining Artificial Technology
At its core, artificial technology refers to the broad spectrum of innovations designed to simulate human intelligence. This includes systems that can learn, reason, and respond to stimuli, often in ways that mimic human thought processes. The term encompasses various subfields like machine learning, computer vision, and neural networks, each contributing to a broader understanding of how technology can work in harmony with human capacities.
To break it down, one might say:
- Machine Learning: Algorithms that improve through experience.
- Natural Language Processing: Enabling machines to understand and generate human language.
- Robotics: Physical manifestations of AI, creating machines that can perform tasks autonomously.
These elements work together, forming a framework that allows us to push boundaries and achieve things that were once deemed impossible.
Historical Context and Evolution
The roots of artificial technology stretch back decades, with early imaginings of machines capable of thought. It was not just science fiction; figures like Alan Turing laid the groundwork with the Turing Test, questioning what it means for machines to "think." Fast forward to the 1950s and 60s, the field saw its first real sparks of life with simple AI programs that could solve algebra problems or play games like chess.
As computer technology advanced, so did the capabilities of artificial tech. The integration of big data and increased computational power in the 21st century birthed a new era. Suddenly, AI systems could analyze intricate data patterns, leading to breakthroughs in diverse fields such as healthcare and finance.
One significant point in this evolution was the development of deep learning in the 2010s, which allowed for astonishing strides in tasks like image recognition and language translation. Now, we have AI such as OpenAI's ChatGPT and IBM's Watson that can not just process information but also understand context and nuance, pushing us closer to truly intelligent systems.
"The progress of artificial technology today is reminiscent of the early days of the internet; we are still figuring out how to maximize its potential."
As we reflect on this historical context, it becomes clear that the journey of artificial technology wasnât linear but filled with innovative leaps and setbacks. What's yet to come remains, in many ways, an open book waiting for subsequent chapters to be written as these technologies continue to evolve and integrate into our daily lives.
Core Components of Artificial Technology
Artificial technology is built on several essential components that form the bedrock of its capabilities and applications. Understanding these core elements not only sheds light on how artificial technology operates but also highlights its benefits and the considerations that come with its use.
Machine Learning Algorithms
Machine learning algorithms serve as the arteries of artificial technology. They enable systems to learn from data without explicit programming. By processing vast amounts of information, these algorithms can discern patterns and make predictions. For students and professionals, grasping how these algorithms work is critical since they lay the foundation for applications in various fields such as finance, healthcare, and marketing.
- Types of Algorithms:
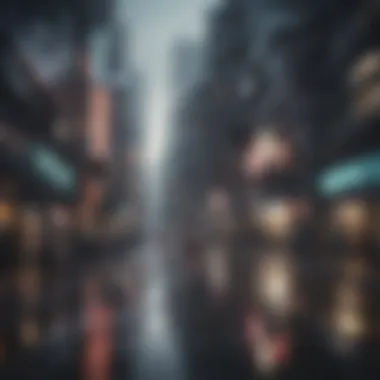
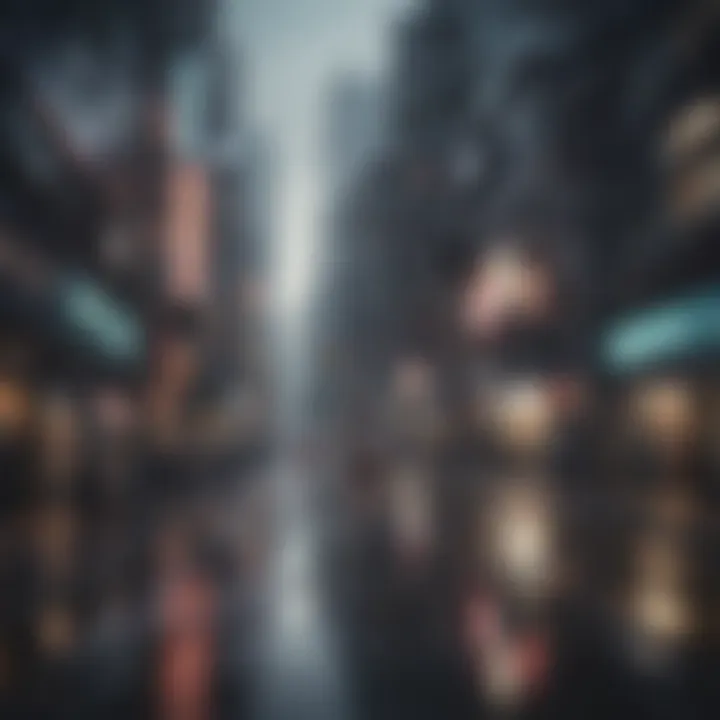
- Supervised Learning: Learns from labeled data.
- Unsupervised Learning: Identifies patterns without pre-existing labels.
- Reinforcement Learning: Learns through trial and error, making it particularly effective in dynamic environments.
With intense automation in industries, the utility of machine learning algorithms has skyrocketed, transforming conventional practices into data-driven decisions. As these algorithms evolve, ethical implications, particularly regarding bias and discrimination, also come to the forefront. It's vital to employ these algorithms responsibly to facilitate positive societal impacts.
Neural Networks and Their Functionality
Neural networks mimic the human brain's functioning, allowing machines to recognize patterns and make informed decisions. This remarkable adaptability makes them a key player in the realm of artificial technology. Each layer in a neural network extracts features from the data, progressively leading to deeper insights.
- Architecture: A neural network is structured in layers, including:
- Input Layer: Receives the initial data.
- Hidden Layers: Where data processing occurs.
- Output Layer: Delivers the final decision or prediction.
Neural networks find their significance in areas like image processing, natural language understanding, and complex simulation tasks. They can refine their outputs as they consume more data, aptly illustrating how the core components of artificial technology intricately interlace with learning and intelligence.
Natural Language Processing Techniques
Natural Language Processing (NLP) is essential for enabling machines to understand and interact with human language, bridging the communication gap between humans and technology. It encompasses a myriad of techniques ranging from syntax analysis to sentiment evaluation.
Some key aspects of NLP include:
- Tokenization: Breaking down text into meaningful units.
- Named Entity Recognition: Identifying entities within text, such as names, dates, and locations.
- Sentiment Analysis: Gauges the emotional tone behind a body of text.
The importance of NLP has surged in recent times as conversational agents and AI-driven chatbots have become prevalent. As organizations strive to enhance user interactions, NLP plays a pivotal role in making these technologies intuitive and beneficial.
"In the realm of artificial technology, the ability to understand and generate human language fundamentally alters the landscape of communication."
These core components intertwine to create a robust framework that underpins artificial technology. Each component, from machine learning algorithms to neural networks and natural language processing, contributes to a more sophisticated, responsive, and effective technological environment. The intrigue lies not only in their current functionalities but also in their potential for future innovations.
Applications Across Disciplines
The topic of applications across various disciplines greatly illuminates the relevance of artificial technology in today's landscape. There are numerous fields where the integration of artificial tech is not just a perk, but a game changer. Understanding the diverse uses can help in recognizing the potential benefits and challenges that come along with it. As we peel back the layers, it's essential to note that each application has its own distinct elements that bring something valuable to the table.
Healthcare Innovations
Telemedicine
Telemedicine stands as a crucial advancement in the healthcare sector. It leverages technology to connect patients with healthcare providers via virtual visits. The key characteristic here is accessibility, allowing individuals in remote areas to receive care without the need for extensive travel. This becomes especially beneficial during times of crisis, like a pandemic, when traditional hospital visits can pose health risks. However, telemedicine does face challenges; issues such as bandwidth limitations in rural areas can interrupt consultations. Moreover, patients may worry about the privacy of their medical information, which needs thorough attention.
Predictive Analytics for Patient Care
Predictive analytics for patient care sets a new horizon in how healthcare professionals manage and administer treatments. By utilizing algorithms to analyze medical data, this approach identifies potential health risks even before they manifest. The standout feature here is the ability to customize care based on data-derived insights. For instance, analyzing a patient's history might spark action to preemptively treat chronic conditions. Yet, while predictive analytics offers many advantages, it also raises questions about the ethical use of data. Wouldn't it be troubling if biases in data lead to suboptimal patient care?
Automation in Industry
Manufacturing Efficiency
Manufacturing efficiency is a cornerstone of competitive business practices today. The specific aspect of this is found in fine-tuning production lines through automation with artificial technology. No one can ignore how robots take over repetitive tasks, streamlining production and reducing human error. This brings down costs and ramp up output, thereby making it a popular choice among industrial players. However, reliance on technology can occasionally create complications, like potential job loss for workers who might find themselves replaced by machines. Bridging that gap is key to a successful transition.
Supply Chain Optimization
Optimizing supply chains is critical for businesses striving to thrive. Here, artificial technology functions like a maestro, coordinating the myriad elements involved in the supply chain. This capability allows firms to respond swiftly to changes in demand and minimize waste, underscoring its significance in today's fast-paced market. However, with these advantages come concerns about data security and the reliance on technology that could be vulnerable to cyber threats. It becomes evident that the benefits must be weighed against the risks.
Artificial Technology in Education
Adaptive Learning Systems
Adaptive learning systems are increasingly gaining traction in educational settings. The allure lies in their capacity to personalize learning experiences based on studentsâ specific needs, thereby enhancing overall educational outcomes. They adjust difficulty levels and suggest resources in real-time, reflecting a tailored approach. While effective, one must remain cautious. There is a risk that heavy reliance on such systems may inadvertently overlook the importance of human interaction in learning. Balancing technology with personal engagement is essential.
Data-Driven Insights for Teaching
Data-driven insights for teaching may seem like just numbers and charts, but they are vital for shaping effective educational strategies. This specific aspect looks at how empirical data informs educators about student performance and engagement. By examining these insights, teachers can pull from a treasure chest of information to improve methods and materials. On the downside, a heavy focus on quantitative metrics might overshadow qualitative experiences that often provide a fuller picture of student learning. Hence, the integration of data needs a thoughtful approach.
"Understanding the intricacies of artificial technology applications across diverse fields is essential not only for leveraging benefits but also for addressing inherent challenges."
Ethical Considerations
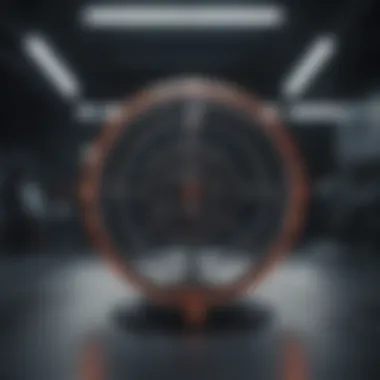
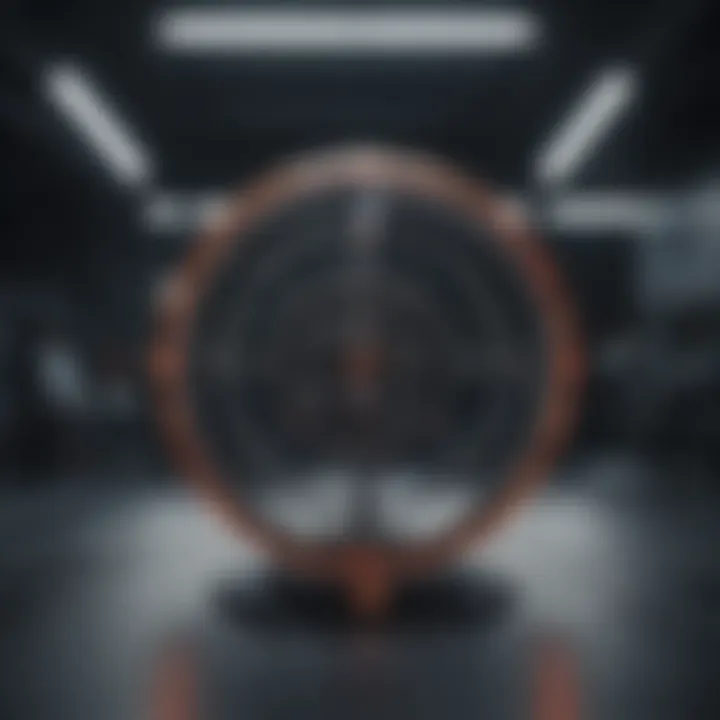
In the realm of artificial technology, ethical considerations play a pivotal role that goes beyond mere function and innovation. The implications of how artificial technologies interact with society raises important queries that must be tackled head-on. Understanding these ethical dimensions is crucial, as it helps navigate the balance between technological advancement, societal impact, and individual rights. As we step into a future intertwined with artificiality, examining ethical concerns is not just necessaryâitâs paramount.
Bias and Fairness in Algorithms
Algorithms often serve as the backbone of various artificial technology implementations. However, these algorithms can contain biases that reflect historical inequalities. A case in point is the facial recognition technologies that have exhibited higher error rates for people of certain ethnic backgrounds. This shortfall can lead to unfair outcomes, reinforcing stereotypes and perpetuating systemic biases. Itâs critical to scrutinize the training datasets used in these algorithms, ensuring they are diverse and comprehensive.
Addressing bias in algorithms not only enhances fairness but also builds trust in artificial technologies. The following steps can help improve bias mitigation:
- Diverse Data Collection: Gathering data across different demographics to ensure representation.
- Regular Audits: Periodically assessing algorithms to identify and rectify bias.
- Transparent Documentation: Keeping a clear record of how algorithms were developed and trained.
"The challenge isnât just about eliminating bias; itâs about fostering fairness through technology."
Privacy Concerns with Data Usage
Privacy is another significant concern in the realm of artificial technology. The constant collection of personal data raises questions about how that information is used, who has access to it, and what safeguards are in place to protect it. As users engage with various technologies, they often leave behind digital footprintsâdata that can be exploited if not handled properly.
To safeguard privacy, several approaches can be implemented:
- Data Anonymization: Ensuring that collected data cannot be traced back to individual users.
- User Consent: Requiring clear, informed consent for data collection and usage.
- Transparent Policies: Organizations must communicate how data is utilized, stored, and shared.
By fostering a culture of privacy awareness and responsibility, businesses can build consumer confidence in their use of artificial technologies.
Regulations and Compliance
As artificial technologies increasingly integrate into daily life, regulations become essential to guide their use responsibly. Regulatory frameworks dictate how data should be collected, stored, and processed, ensuring compliance with legal standards. Organizations must keep abreast of existing laws, such as the General Data Protection Regulation (GDPR) in Europe, which emphasizes user rights and data protection measures.
Implementation of effective regulations involves several key actions:
- Regular Reviews: Keeping policies current with evolving technologies to address new risks.
- Stakeholder Engagement: Involving diverse voicesâincluding technologists, ethicists, and community representativesâin developing regulations.
- Responsibility and Accountability: Establishing clear lines of responsibility for organizations in their data handling practices.
By prioritizing regulatory compliance, organizations can not only avoid potential legal issues but also demonstrate their commitment to ethical practices in artificial technology.
The Future of Artificial Technology
The realm of artificial technology stands on the precipice of extraordinary possibilities and profound challenges. Its trajectory is influenced by emerging trends, ethical dilemmas, and the essential role of human oversight. Understanding the future of this field not only helps in recognizing its potential benefits but also in addressing the myriad implications it may have across various sectors. This section will explore these specific elements, focusing on how they intertwine to craft the narrative of tomorrow's technological landscape.
Trends and Innovations to Watch
Forecasting the direction artificial technology may take can feel like trying to predict the weather weeks in advance. However, certain patterns and innovations are beginning to emerge as bellwethers of change. From improved machine learning capabilities to the expansion of conversational agents, the horizon is dotted with game-changing advancements. Here are some key trends that one should keep an eye on:
- Federated Learning: This innovative approach allows models to learn from decentralized data without sharing sensitive information. It could elevate privacy standards across applications.
- Explainable AI (XAI): As reliance on AI grows, so does the need for transparency in decision-making. XAI aims to demystify how algorithms arrive at conclusions.
- Quantum Computing Integration: This emerging technology could drastically speed up computational tasks, providing deeper insights in sectors such as finance and healthcare.
- Generative Models: Techniques like DALL-E and ChatGPT exemplify how AI can generate novel content, which poses questions about creativity and originality in art and writing.
These advancements could very well redefine industries, reshaping how businesses and individuals interact with technology.
Potential Risks and Challenges
While the future is ripe with excitement, it's equally peppered with potential risks that merit serious contemplation. Without careful management, some trends could backfire, leading to unintended consequences. Key challenges to consider include:
- Data Privacy Issues: As AI relies heavily on data gathering and analysis, there are valid concerns regarding privacy breaches. Striking the right balance between utility and confidentiality remains crucial.
- Job Displacement: Automation, driven by AI innovations, has the potential to replace many traditional jobs. Upskilling and reskilling the workforce will be pivotal to mitigating this impact.
- Ethical and Moral Considerations: Algorithms can inadvertently perpetuate biases inherent in training data. Developing fair and unbiased AI systems must be a priority.
- Dependency on Technology: An overreliance on AI may lead to diminished human oversight and critical thinking abilities. This could cultivate a scenario where technology inadvertently undermines human decision-making.
The Role of Human Oversight
Human oversight acts as the anchor in the turbulent waters of AI advancements. While machines can process vast amounts of information and even perform complex tasks, the human element is irreplaceable when it comes to ethical considerations and contextual understanding. Key aspects include:
- Collaborative Intelligence: Rather than viewing AI and humans as opposing forces, the focus should shift towards collaboration, where each complements the other's strengths.
- Ethics in Deployment: Human oversight ensures that ethical frameworks guide the implementation of AI technologies, fostering trust among users and stakeholders.
- Skill Development: Continuous training programs will enable individuals to effectively work alongside AI systems, embracing a future where technology enhances human skill rather than replaces it.
- Accountability Frameworks: Establishing clear accountability mechanisms for AI decisions can mitigate risks and address concerns related to bias, transparency, and privacy.
"As we stand at the crossroads of possibility, it is crucial to remember that the evolution of technology must align with humanityâs ethical compass."
Case Studies of Artificial Technology Implementation
Case studies serve as a powerful lens through which we can observe the real-world application of artificial technology. They bring to light the intricacies of implementation, showcasing both successes and hurdles faced by different organizations. The importance of this topic lies not just in sharing what worked or didn't, but in drawing lessons that go beyond the immediate context. Each case can illuminate paths forward in a diverse array of sectors, from business to healthcare, providing insights that can inform future endeavors in artificial technology.
Successful Deployments in Business
The business realm has seen numerous successful implementations of artificial technology, revolutionizing operational practices and customer engagements alike.
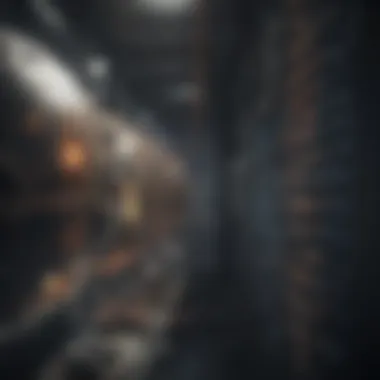
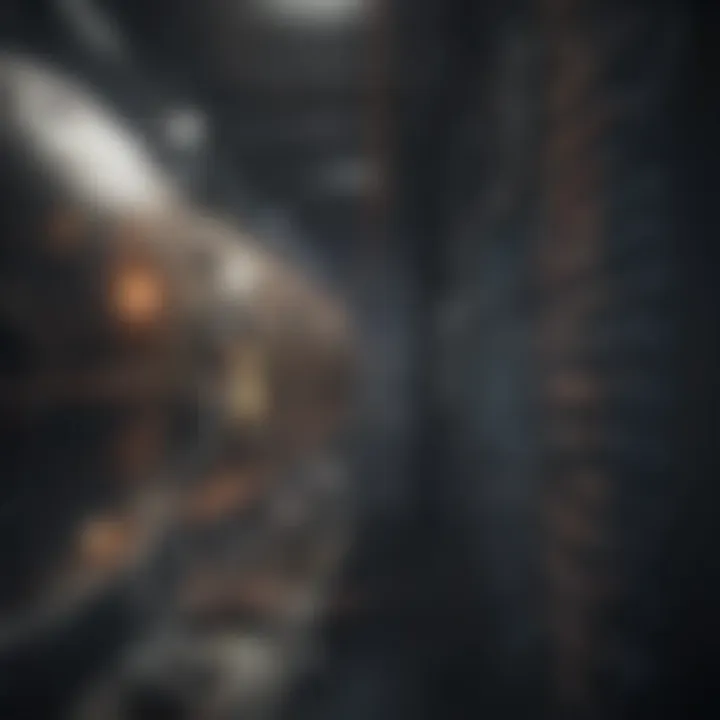
One remarkable example comes from Netflix, which employed sophisticated algorithms to optimize user experience through personalized content recommendations. By analyzing viewing habits, the company tailors suggestions, enhancing subscriber engagement and retention. This method not only boosts user satisfaction but also translates into significant financial benefits.
Another illustrative case is that of Amazon. Leveraging robotics for warehousing, they have streamlined logistics, resulting in faster delivery times. Robots pick items and fulfill orders much more rapidly than human workers could, allowing Amazon to meet the growing demands of online shopping effectively. This deployment has reshaped not only their operational model but also set new standards for the entire e-commerce industry.
Moreover, General Electric has harnessed artificial intelligence-driven predictive maintenance. By analyzing data from machinery, they predict failures and schedule maintenance proactively, leading to reduced downtime and extensive cost savings.
The benefits of these successful deployments range from improved efficiency and customer satisfaction to belting out cost reductions. However, as with all stories of success, itâs essential to ask: What can we learn from these implementations?
Challenges Faced by Organizations
Despite the advantages that artificial technology can offer, the road to implementation is not always smooth. Organizations can encounter various challenges that require careful navigation.
For instance, a case involving a major telecommunications company illustrates the complexities of integrating AI into existing systems. The organization faced hurdles with data silosâdepartments hoarding their insights rather than sharing them. This limited the efficacy of machine learning models, showing that collaboration among teams is crucial.
Another example can be found in the healthcare sector where an institution aimed to integrate AI for patient diagnostics. Initial enthusiasm quickly got tempered by issues like data privacy and regulatory compliance. Navigating HIPAA regulations posed serious concerns over patient data handling, ultimately requiring extensive legal reviews and changes in approach.
In addition, the issue of workforce adaptation cannot be overlooked. Employees may resist alterations to established routines or feel threatened by automation. A manufacturing company found that without proper training programs, the introduction of robots led to job dissatisfaction and confusion among staff, affecting overall productivity.
Engaging with these challenges is essential for any organization looking to implement artificial technology successfully. A thoughtful approach, emphasizing collaboration, compliance, and continuous training, can mitigate risks while maximizing potential benefits.
"The deployment of artificial technology is a journey, not a destination. Every case teaches us something new, shaping the narrative of our future strategies."
Cross-Disciplinary Insights
Artificial technology is not solely a realm of computer science or engineering; it is a multifaceted sphere that intersects with other fields, fostering a fertile ground for innovation and ethical discourse. This cross-disciplinary nature adds depth to understanding artificial technologyâs implications. By weaving together insights from various disciplines like neuroscience and philosophy, we equip ourselves to navigate the complexities inherent in technological advancement. Each discipline offers unique perspectives, enhancing our critical thinking and expanding our comprehension of this rapidly-evolving field.
Intersections with Neuroscience
The interplay between artificial technology and neuroscience is a particularly fascinating area. Neural networks, a significant aspect of artificial intelligence, draw inspiration from the human brain's structure and functionality. Understanding how the brain processes information can help refine the development of these networks, leading to more sophisticated and efficient AI systems.
Consider the way neural pathways operate in our brains to handle language, recognize faces, or respond to stimuli. These insights not only inform algorithm design but also push boundaries in areas like cognitive computing and machine learning. For example, by examining patterns in brain function, researchers can uncover ways to enhance natural language processing models, enabling machines to better understand and produce human-like language.
Moreover, neuroscience provides ethical considerations, especially when it comes to the implications of AI in mental health. Systems designed to analyze emotional states or cognitive functions must be built with a deep understanding of the neurobiological underpinnings of behavior, ensuring that they respect individual differences and vulnerabilities.
The Influence of Philosophy in AI Discussions
Philosophy plays an undeniable role in shaping discussions around artificial technology. It raises essential questions regarding consciousness, free will, and the moral implications of creating machines that can think or act autonomously. The dichotomy between machine intelligence and human intellect is a hotbed of philosophical debate; discussions about what it means to be sentient and the ethical treatment of AI beings arise continually.
Incorporating philosophical perspectives allows us to probe deeper into the consequences of artificial intelligenceâs integration into everyday life. For instance, the trolley problemâan ethical dilemma that deeply examines moral decisionsâhas emerged in discussions of autonomous vehicles. Developers must contend with programming ethical frameworks into AI systems to navigate complex decisions, ultimately reflecting human values and ethics.
"Without a solid philosophical foundation, technological advancement may lead us astray, prioritizing efficiency over ethical responsibility."
The fusion of philosophy with artificial technology not only encourages a more comprehensive consideration of its impacts but also fosters a culture of responsible innovation. This collaboration ensures that as we stride into the future, we remain mindful of the principles and ethics that ought to guide us, preventing careless detours on our journey into the realm of AI.
Through the investigation of these intersections, it becomes clear that a multidisciplinary approach to artificial technology enriches our understanding and equips us to better deal with the challenges and opportunities it presents.
Closure
As we wrap up this exploration into the realm of artificial technology, it is vital to reflect on the significance of not just the innovations that have transformed countless industries, but also the ethical implications that accompany these advancements. The myriad benefits of artificial technology, from improved efficiency in healthcare to enhanced learning in education, cannot be understated. However, alongside this progress, we must remain cognizant of the potential pitfalls and moral dilemmas that may arise.
Recapping the Evolution of Artificial Technology
Artificial technology has traversed a remarkable journey since its inception. Initially rooted in rudimentary algorithms, the field has evolved into a sophisticated landscape characterized by machine learning, deep learning, and extensive data analytics. Looking back at milestones such as the development of the perceptron in the 1950s to the rise of natural language processing in recent years, one can appreciate just how far the discipline has come.
Key elements in this evolution include:
- Algorithmic Advancements: The transition from simple rule-based systems to complex neural networks.
- Integration with Big Data: The synergy between machine learning and vast datasets has propelled capabilities in predictive analytics and automation.
- Interdisciplinary Collaborations: Fields like neuroscience and psychology have contributed insights, further enriching AI's development.
As we look at these facets, it becomes clear that the evolution of artificial technology is not merely technical; it is a reflection of our changing societal needs and aspirations.
Looking Ahead: Future Perspectives
The future of artificial technology is brimming with potential yet laden with challenges. Emerging trends such as quantum computing, further integration of AI in everyday applications, and the increased push for ethical AI implementation indicate where the field may head.
Below are a few forward-looking elements to consider:
- Enhanced Human-Machine Collaboration: As machines become more adept at certain tasks, the aim will be to find a balance that plays to the strengths of both humans and AI.
- Ethical AI Development: Ensuring that policies are in place to reduce bias and safeguard privacy will be critical as technology evolves.
- Global Impact of AI: The ramifications of AI advancements will not be confined within borders; the implications on a global scale will shepherd discussions about governance and equitable access.
"Navigating the future of artificial technology involves not just technological mastery, but also a commitment to thoughtful stewardship of its applications."
By weaving together these considerations, we reinforce an understanding that while artificial technology holds promise, its future must be shaped by careful, inclusive, and ethical deliberation.