AI in Genetics: Revolutionizing Genomic Research
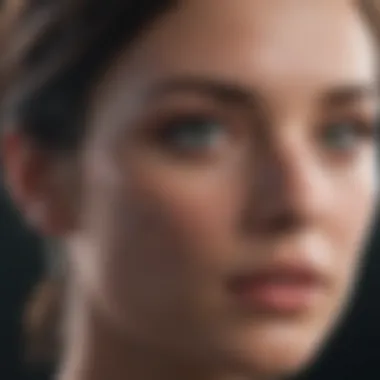
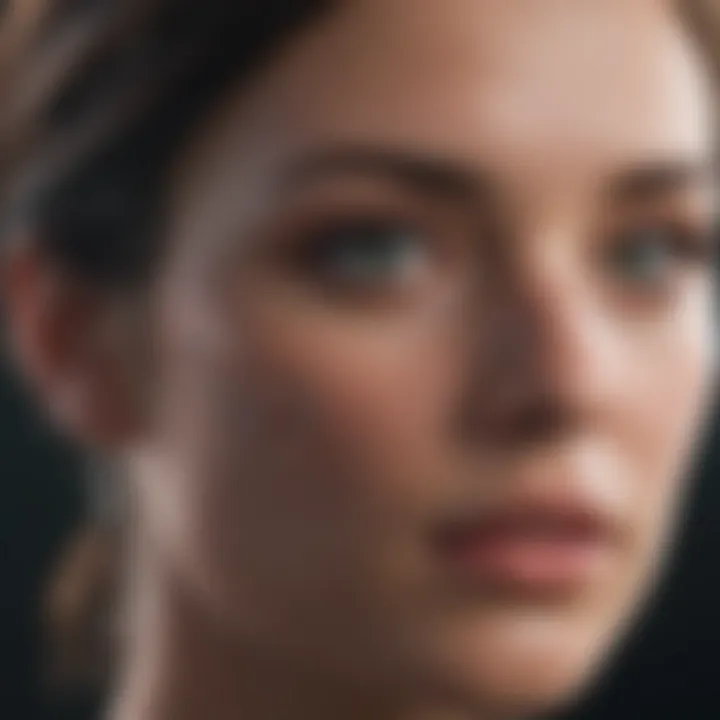
Intro
Artificial intelligence (AI) is reshaping many domains, but few fields are experiencing as profound a transformation as genetics. The confluence of AI with genomic research brings forth innovative methodologies that enhance analysis and understanding of genetic data. By utilizing machine learning algorithms and sophisticated data analytics, researchers are poised to delve deeper into genetic structures and their implications for health.
As the landscape of genomic research evolves, it becomes essential to explore AI's role in advancing genetic studies and precision medicine. Consequently, this article will discuss the engagement of AI in pivotal processes, such as gene editing, disease diagnosis, and personalized treatment strategies. Additionally, considerations regarding the ethical landscape surrounding these technologies will be addressed.
Understanding these factors is paramount, as AI applications may lead to groundbreaking discoveries in deciphering the intricacies of complex genetic diseases and traits.
Prologue to Artificial Intelligence in Genetics
The integration of artificial intelligence (AI) in genetics is reshaping how researchers approach genomic studies. Understanding the significance of AI in genetics is vital for grasping the broader implications of genomic research. AI brings forth a paradigm shift in research methodologies, enabling enhanced data analysis, predictive modeling, and pattern recognition in complex genetic datasets. The ability to process vast amounts of information swiftly unlocks new potential in studying genetic diseases and traits. Through this introduction, we will explore specific elements, benefits, and considerations regarding the intersection of AI and genetics.
Defining Artificial Intelligence
Artificial Intelligence refers to the simulation of human intelligence in machines designed to think and learn like humans. AI technology encompasses various methodologies, including machine learning and neural networks. These systems analyze data, identify trends, and make decisions based on patterns. In genetics, applying AI allows for the automation of repetitive tasks, leading to more efficient research processes. This efficiency is essential, given the ever-growing volume of genetic data generated by modern sequencing technologies. AI's capacity to enhance predictive accuracy can significantly impact genetic research, especially when exploring the genetic basis of complex diseases.
The Intersection of AI and Genetics
The fusion of AI and genetics creates a synergy that propels genomic research forward. AI algorithms can analyze genetic sequences, identify mutations, and predict their impact on health. For example, machine learning models can discern subtle differences in gene expression that may indicate various conditions. This capability opens avenues for precision medicine, enabling treatments tailored to individual genetic profiles. Moreover, by harnessing big data, researchers can uncover correlations between genetics and environmental factors, fostering a holistic understanding of gene-environment interactions.
"AI provides the tools necessary to decipher the complexities of the genome, making it easier to uncover the underlying mechanisms of diseases."
As genomic data expands, the collaboration between AI and genetics deepens. An intricate interplay emerges, wherein AI not only assists in conducting research but also incubates innovative approaches to unravel the mysteries of genetics. In turn, this evolution culminates in improved diagnostic methods and personalized treatment plans, emphasizing the crucial role of AI in shaping the future of genetics.
Historical Context
Understanding the historical context of artificial intelligence in genetics is vital. It provides a foundation to appreciate how far the field has progressed and the significant changes AI has brought about in genomic research. The evolution of genetic studies has been marked by various milestones that have set the stage for today’s advanced methodologies utilizing AI. This section will elaborate on key developments, emphasizing their relevance in shaping current research practices and future applications.
Early Developments in Genetic Research
The beginning of modern genetics can be traced back to the early 20th century when Gregor Mendel's foundational work on inheritance patterns was rediscovered. This period laid the groundwork for genetic science. As researchers began to understand DNA's structure, particularly following Watson and Crick's double helix model revelation in 1953, the field moved dramatically forward. The first sequencing of a gene marked a turning point, allowing for deeper exploration into genetic functions and relationships.
Before long, genetic research expanded beyond individual genes. The Human Genome Project, initiated in the 1990s, aimed to sequence the entire human genome. This objective ultimately required advanced computational tools to process the vast amounts of data generated. The endeavor highlighted the increasing complexity and scale of genetic studies, making it clear that traditional methods were insufficient for managing and interpreting genomic information.
This historical backdrop shows how early achievements in genetics set the stage for the integration of more sophisticated technologies like AI. Researchers faced with abundant data needed solutions for analysis, which paved the way for computational approaches that would later incorporate AI.
Emergence of Computational Tools
As genetic research advanced, the need for computational tools became crucial. The comparison of genetic sequences and the identification of specific mutations required sophisticated technologies. The emergence of bioinformatics in the late 20th century played a critical role. It offered methods for analyzing complex biological data, leveraging computer technology to interpret genetic information.
Key software developed during this time, such as BLAST for DNA sequence alignment, illustrated early efforts to harness computational power in genetics. As datasets expanded, these tools evolved. The incorporation of algorithms allowed for more precise anomaly detections and predictive modeling of genetic traits.
With the rise of big data analytics in the 2010s, computational tools became more integrated into genetic research workflows. This shift resulted in the ability to analyze vast amounts of genetic information rapidly, enabling researchers to uncover patterns that were previously invisible. Consequently, the integration of machine learning began to emerge as a front-runner in genetic research. This synergy marked a new phase in the interplay between genetics and technology, directly influencing the abilities of researchers to unravel the complexities of genetic diseases and development patterns.
Machine Learning in Genomics
Machine learning is pivotal in genomics, enhancing data analysis and application development. It enables researchers to uncover patterns that are not easily identifiable through traditional methods. The vast amount of genetic data generated by sequencing technologies demands advanced analytical tools for proper interpretation. Thus, machine learning helps to accelerate discoveries in genetic research, paving the way for more precise and personalized medical interventions.
Types of Machine Learning Algorithms
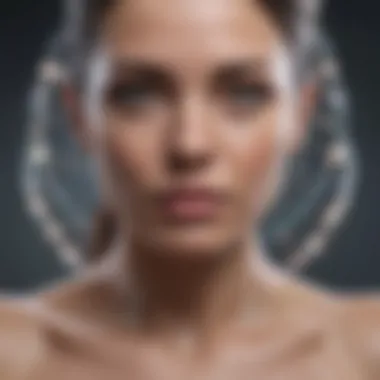
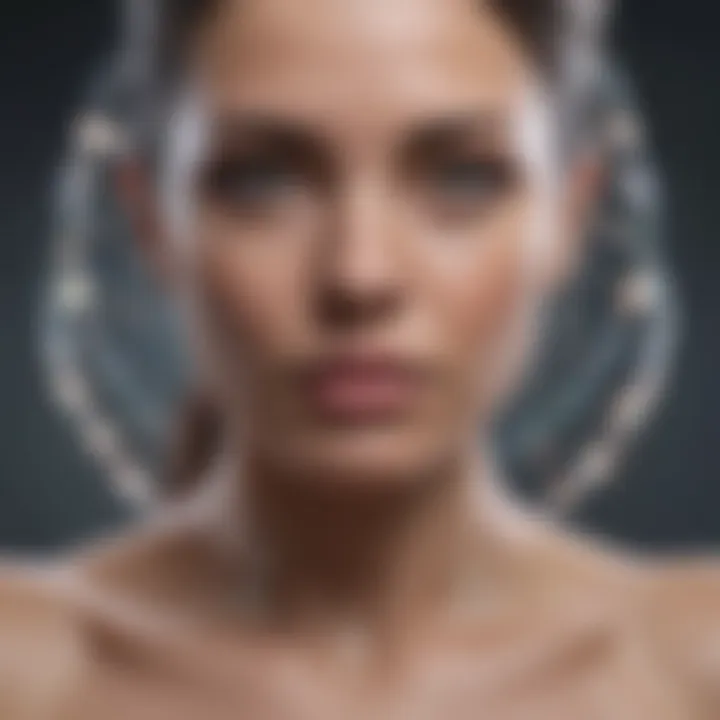
Supervised Learning
Supervised learning is one of the most utilized machine learning techniques in genomics. It involves training algorithms on labeled data, where the outcome is known. This feature allows the model to make predictions based on new, unseen data. Its primary contribution is in enhancing the accuracy of diagnostic models for genetic disorders. Supervised learning is popular due to its effectiveness in classification tasks, such as identifying variants associated with specific diseases. However, it requires a substantial amount of high-quality labeled data, which can be a limitation in some genomic studies.
Unsupervised Learning
Unsupervised learning is another essential algorithm in the realm of genomics. Unlike supervised learning, it works with unlabeled data, allowing the algorithm to identify patterns without prior information. This is particularly beneficial for exploring genetic variability and discovering new subtypes of diseases. The ability to cluster data points provides insights into the underlying genetic architecture of various conditions. However, interpreting the results from unsupervised learning can be challenging since there are no definitive labels to guide the analysis.
Reinforcement Learning
Reinforcement learning is a less common but intriguing approach in genomics. It functions on the principle of learning through interactions and feedback from the environment. In context of genetics, it can optimize treatment protocols or gene editing strategies by continuously evaluating the consequences of various actions. Its main strength is the ability to make sequential decisions that adapt to the environment. However, the complexity of genetic landscapes can make reinforcement learning computationally intensive and time-consuming.
Applications of Machine Learning in Genetic Analysis
The applications of machine learning in genetic analysis are broad and impactful. They range from facilitating gene discovery to enhancing genomic prediction. Machine learning models can analyze large datasets, identify disease-associated variants, and even predict patient responses to treatments.
Key applications include:
- Variant Annotation: Classifying genetic variants based on their potential impact on health.
- Disease Phenotyping: Utilizing machine learning to categorize patients based on genetic information and clinical data.
- Predictive Modeling: Forecasting disease risk based on genetic markers and environmental factors.
- Targeted Drug Development: Aiding in the identification of genetic targets for therapeutic interventions.
Data Analysis in Genetics
Data analysis has become a cornerstone in the field of genetics, particularly with the influx of data generated from various genomic technologies. The rapid advancement in sequencing technologies has resulted in extensive datasets that require sophisticated analytical techniques. Analyzing this data enables researchers to derive meaningful insights into genetic structures and functions. This ensures that scientists can identify correlations, patterns, and anomalies that may not be evident through traditional methods.
The significance of data analysis in genetics can hardly be overstated. Leveraging artificial intelligence improves not only the efficiency of processing these massive datasets, but also the accuracy of the conclusions drawn. By integrating AI with genomic research, improvements in predictive modeling and statistical analysis can be achieved.
Role of Big Data in Genetic Research
Big data plays a crucial role in advancing genetic research. The wealth of genetic information that exists today is largely due to high-throughput sequencing technologies, such as Next Generation Sequencing (NGS). These technologies have made it possible to sequence entire genomes swiftly and at a reduced cost. However, this abundance of data presents challenges. Managing and analyzing such extensive information requires robust computational tools.
Utilizing big data analytics allows researchers to:
- Identify genetic variations that might be linked to diseases.
- Enhance understanding of complex traits through large-scale studies.
- Facilitate the discovery of new biomarkers associated with particular conditions.
AI methodologies help to tackle these challenges, yielding insights that were previously unattainable. By applying algorithms that can learn from the data, researchers can discern patterns that lead to significant breakthroughs in genomics.
Data Mining Techniques and Their Impact
Data mining techniques are essential in genetics, particularly when it comes to extracting valuable information from large datasets. These techniques incorporate a range of methodologies, including classification, clustering, and regression analysis. The efficacy of these techniques in genetic research fosters deeper insights into genomic data.
Among the most impactful data mining techniques are:
- Classification algorithms which categorize genetic sequences based on known features.
- Clustering methods that group similar genetic traits, making it easier to study them as a whole.
- Regression analysis that provides a way to predict outcomes based on various genetic markers.
The integration of these mining techniques results in:
- Enhanced capability to correlate specific genetic features with diseases.
- Improved genetic risk assessments, leading to better preventative strategies.
- Insights into population genetics, helping to understand how traits and diseases distribute across different groups.
"The application of data mining in genetics not only streamlines the research process but also enhances the overall quality of discoveries."
As the field of genetics evolves, the importance of effective data analysis will continue to grow, fundamentally altering how genetic information is studied and utilized.
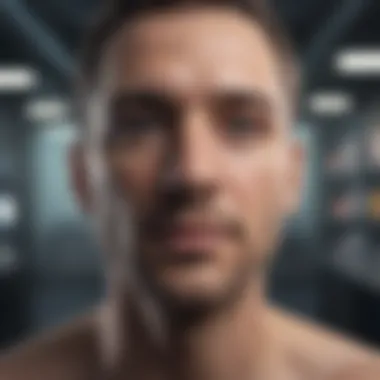
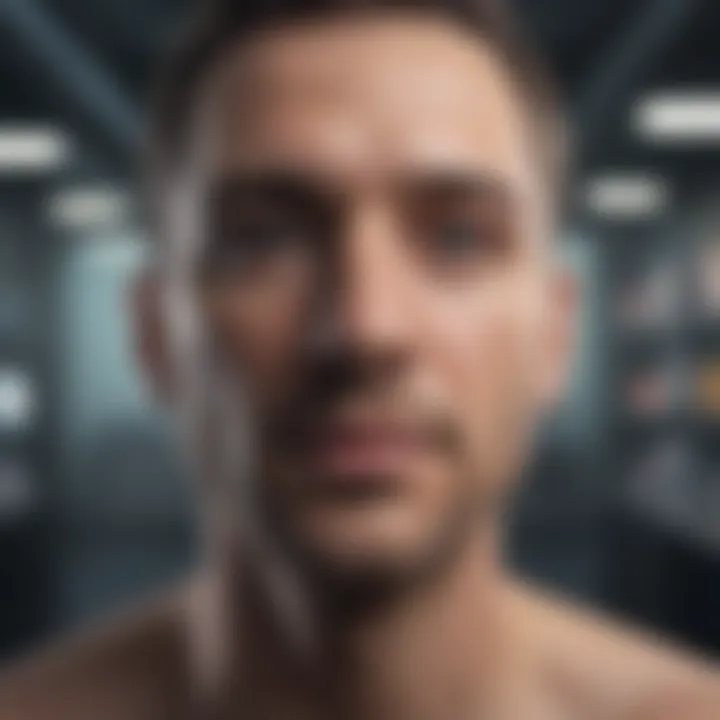
Gene Editing Technologies
Gene editing technologies represent a groundbreaking aspect of genetic research, fundamentally altering how scientists approach the study of genes and their functions. One significant method within this realm is CRISPR. It provides not only precision but also efficiency in editing genetic material. This technology enhances our ability to understand genetic disorders, develop treatments, and even make improvements in agriculture.
The integration of AI with gene editing strengthens predictive capabilities. By analyzing large datasets, AI identifies potential off-target effects and optimizes the editing process. Thus, researchers can reliably achieve desired outcomes while minimizing unintended consequences. AI's influence on gene editing enhances both the scope and safety of genomic experiments, marking a pivotal revolution.
CRISPR and AI Advancements
CRISPR, short for Clustered Regularly Interspaced Short Palindromic Repeats, has transformed how genetic manipulation is conducted. Its ability to quickly and accurately target specific genes is unmatched. Recent advancements have only amplified its potential.
Combining CRISPR with artificial intelligence has opened new avenues in the field. AI algorithms analyze genomic data, predicting which segments of DNA should be targeted for editing. These models utilize vast amounts of previous research, enhancing the efficiency of CRISPR applications. AI can also simulate potential outcomes after gene editing, allowing researchers to anticipate challenges.
Utilization of AI in CRISPR research promotes developments in therapeutic strategies. This is particularly relevant for conditions such as cystic fibrosis and certain cancers. The ability to customize gene editing opens possibilities for personalized therapies tailored to individual genetic makeup.
Ethical Implications of Gene Editing
While gene editing technologies like CRISPR hold immense potential, they also raise ethical considerations that cannot be overlooked. The manipulation of genetic material has societal implications, particularly concerning heritable changes. Gene editing can lead to what some call "designer babies," allowing selection of desirable traits.
The potential for misuse of gene editing technologies demands strict ethical guidelines. Researchers and policymakers must address concerns surrounding equity, access, and unintended long-term effects in ecosystems. The interplay between technological advances and ethical considerations establishes a necessary dialogue focused on responsible innovation.
Accurate ethical frameworks are essential to navigate the risks associated with gene editing and ensure benefits are widespread.
AI in Disease Diagnosis
The integration of artificial intelligence within the realm of disease diagnosis marks a significant pivot in the approach to healthcare and genetics. AI's application in this field enhances our understanding of genetic disorders and improves clinical outcomes. Machine learning techniques enable the analysis of vast data sets, facilitating early diagnosis and personalized treatment strategies for various conditions. As genomics generates an unprecedented amount of data, the necessity for AI to process and interpret this information becomes even more critical. This section explores the nuances associated with predictive techniques in genetic disorders and the integration of AI into clinical decision-making.
Predictive Techniques in Genetic Disorders
Predictive techniques are a cornerstone of AI's role in genetic disorders. These techniques apply algorithms to genetic data to forecast the likelihood of developing a disease based on a person's genetic makeup. The process typically involves several steps: data collection, analysis through machine learning, and result interpretation.
- Data Collection: This involves compiling genomic data, which can include single nucleotide polymorphisms (SNPs) and other genetic variations.
- Machine Learning Algorithms: Supervised learning methods, for instance, leverage labeled data to create models that can predict genetic disorder risks. Algorithms like random forests or support vector machines are employed to sift through patterns within data.
- Interpretation of Results: After analysis, the results need to be communicated clearly to patients and healthcare providers to inform decisions.
The advantages of these predictive techniques are profound. They can aid in identifying predispositions for conditions such as Alzheimer's, cystic fibrosis, or various forms of cancer. By understanding these risks early, targeted prevention strategies can be established, ultimately improving patient care and outcomes.
Integration of AI in Clinical Decision Making
The integration of AI in clinical decision-making represents a transformative shift for genetic healthcare. AI tools offer insights that help healthcare professionals make informed choices regarding diagnosis and treatment. Given the complexity of genetic conditions, AI serves as an essential assistive technology that augments human expertise.
AI systems can analyze a patient’s genetic data alongside their medical history and other relevant factors, leading to a more holistic view. This synthesis of information allows for the development of tailored treatment plans, enhancing precision medicine.
For instance, tools like IBM Watson have been made to assist oncologists in determining the best treatment protocols based on genetic markers found in tumors. This integration ensures that therapies are not only more effective but also potentially reduce adverse effects by aligning treatment closely with the patient’s genetic profile.
Furthermore, AI can continuously learn from new data inputs, which helps improve diagnostic accuracy over time. It can also flag patients who might benefit from genetic testing, thus optimizing resources and ensuring timely intervention.
"AI's role in analyzing complex genetic data highlights its potential for revolutionizing how we approach diagnostics and treatment in clinical settings."
Personalized Medicine and AI
Personalized medicine represents a significant advancement in healthcare, targeting treatment plans based on individual genetic profiles. The integration of artificial intelligence into this field is transformative. AI facilitates the analysis of vast amounts of genetic data to identify specific mutations and diseases associated with these mutations. Through AI, clinicians can create personalized treatment approaches, aiming for higher efficacy and reduced side effects.
In personalized medicine, the role of AI extends to developing treatments tailored to unique patient characteristics. By analyzing large datasets that include genetic variations, patient histories, and response patterns to drugs, AI systems can recommend customized therapy options. This approach not only improves patient outcomes but also streamlines the drug discovery process, making it more efficient.
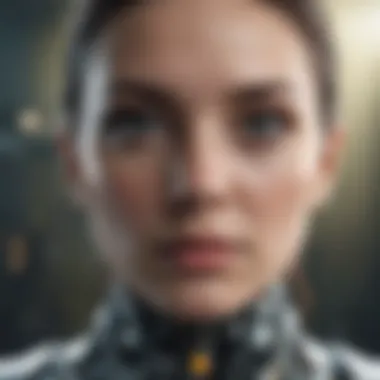
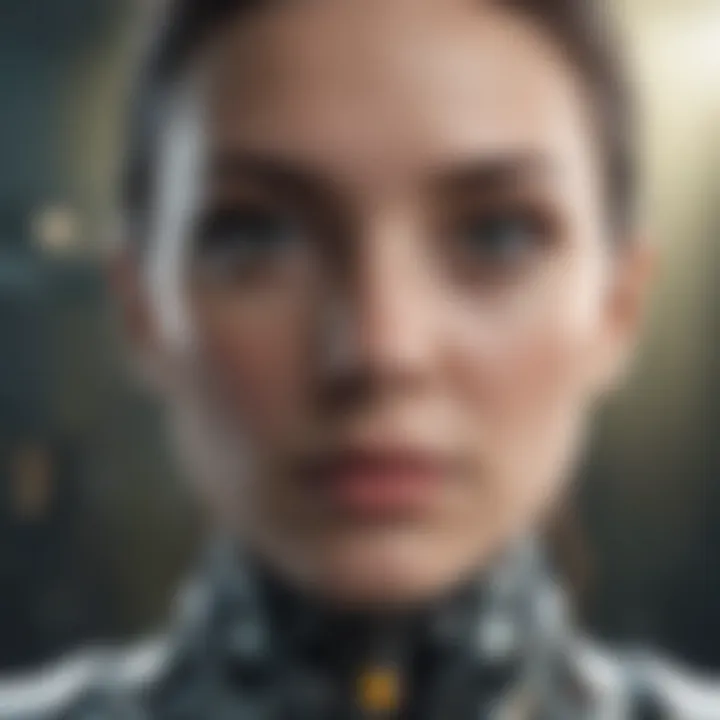
Developing Tailored Treatments
The development of tailored treatments is at the core of personalized medicine. With AI technologies, health professionals can utilize algorithms that sift through numerous variables in patient data. These algorithms can uncover relationships between genetics and treatment responses that may not be evident through traditional analyses.
- Data Integration: AI enables the integration of multiple data sources, including electronic health records, genomic sequencing, and clinical trial results. This comprehensive view is essential for identifying the most appropriate treatments.
- Predictive Modeling: AI models can predict how an individual will respond to specific drugs, which is crucial for conditions such as cancer where treatment responses can vary greatly.
- Optimizing Treatment Plans: AI allows for real-time adjustments to treatment plans as new data becomes available, ensuring that the approach remains optimal throughout the patient's care.
The accurate prediction of treatment efficacy leads to more confident clinical decision-making, which ultimately results in improved health outcomes.
Challenges in Implementation
Despite the promise of personalized medicine powered by AI, several challenges exist in its implementation.
- Data Privacy Concerns: Storing and using personal genetic information raises significant privacy concerns. Safeguarding patient data is paramount.
- Large-Scale Data Requirements: AI systems rely on extensive datasets for training algorithms. Gaps in data can limit the predictive abilities of these systems.
- Interoperability Issues: Different healthcare systems may use varying data formats, complicating the effort to create a unified system that AI can effectively analyze.
- Regulatory Hurdles: The rapid evolution of AI technologies often outpaces existing regulations, which may hinder implementation and innovation in the field.
The journey towards fully realizing the potential of personalized medicine with AI integration may be complex. Getting past these challenges requires cooperation among researchers, healthcare providers, and policymakers.
"In the realm of personalized medicine, AI emerges not merely as a tool, but as a catalyst for redefining therapeutic strategies. It holds the promise of evolving healthcare into a tailor-fitted experience for each patient."
Ethics and Regulations
The integration of artificial intelligence into genetics brings profound implications for ethical standards and regulatory frameworks. As AI technologies redefine how genetic data is processed and utilized, a clear understanding of the ethical considerations and legal structures surrounding this advancement is essential. This section outlines the fundamental ethical issues linked to AI in genetics and the regulatory measures that govern its usage, emphasizing their importance in shaping a responsible and equitable future for genomic research.
Ethical Considerations in AI Utilization
AI's role in genetics raises several ethical dilemmas that researchers, practitioners, and society must confront. Key considerations include:
- Privacy Concerns: Genetic data is inherently sensitive. The potential for AI to analyze vast amounts of personal genetic information can lead to privacy breaches. Ensuring confidentiality while utilizing such data for research is paramount.
- Bias in AI Algorithms: AI systems can inadvertently perpetuate biases present in their training data. This is especially relevant in genetics, where specific populations may be underrepresented. It is vital to ensure that genetic insights derived from AI are equitable and applicable to diverse populations.
- Informed Consent: With advancements in AI, obtaining informed consent from subjects becomes increasingly complex. Participants should fully understand how their genetic data will be used and the potential implications for their privacy and health.
- Accountability: Determining responsibility when AI systems make errors in genetic analysis poses a challenge. Clear guidelines must establish who is accountable—be it researchers, organizations, or the algorithms themselves.
The ongoing dialogue about the ethical implications of AI in genetics must include multidisciplinary perspectives, combining ethics, law, and technology.
Regulatory Frameworks Governing AI in Genetics
To address the ethical concerns associated with AI in genetics, regulatory frameworks are critical. Such regulations guide responsible AI utilization in research, ensuring protections for individuals while fostering innovation. Key frameworks include:
- Health Insurance Portability and Accountability Act (HIPAA): In the U.S., HIPAA provides a framework for protecting sensitive patient information, including genetic data. Adhering to these regulations is vital for compliance when employing AI in medical genetics.
- General Data Protection Regulation (GDPR): In the European Union, the GDPR mandates stringent data protection and privacy standards. This regulation significantly impacts how researchers and organizations use AI to analyze genetic information.
- Ethics Review Boards: Institutional Review Boards (IRBs) require ethics review before research involving human subjects can proceed. These boards assess studies involving AI and genetics to ensure ethical standards remain intact.
- Emerging Guidelines: Organizations like the World Health Organization and various national bodies are developing guidelines specifically for AI and genetics. These emerging regulations help ensure that AI-driven research aligns with societal values and ethical norms.
In summary, addressing ethics and regulations associated with AI in genetics is crucial. As technology advances, so must our efforts to balance innovation with respect for individual rights and social responsibility.
Future Directions
The future directions of artificial intelligence in the field of genetics are crucial as they promise to redefine how researchers approach genomic studies. With advancements in machine learning and big data analytics, the possibilities seem limitless. The integration of AI technology can lead to a better understanding of genetic diseases and facilitate more effective treatments. This section focuses on key elements that highlight the importance of pursuing these directions in genetic research.
- Personalized Medicine: The shift toward personalized medicine is reshaping healthcare. By utilizing AI algorithms to analyze genetic data, researchers can tailor treatments to individual patients. This may enhance patient outcomes significantly compared to traditional methods.
- Genomic Data Interpretation: The complexity of genomic data poses a challenge. AI technologies can process vast amounts of information quickly and accurately, helping researchers uncover valuable insights. Enhanced data interpretation leads to the identification of genetic variations linked to diseases.
- Predictive Modeling: Another future direction involves predictive modeling of genetic disorders. With machine learning, researchers can develop models that predict disease risk based on genetic predisposition. This can provide early intervention opportunities, ultimately reducing disease burden.
Potential Breakthroughs in Genetic Research
AI’s integration into genetic research opens the door to a range of potential breakthroughs. As computational power evolves, researchers are better equipped to tackle questions that were previously seen as insurmountable. Some notable breakthroughs could include:
- Enhanced Accuracy in Gene Mapping: AI can improve the precision of genome-wide association studies (GWAS). This can facilitate the identification of specific genetic variations that influence complex traits.
- Disease Mechanism Elucidation: AI systems programmed with vast datasets could help in understanding the underlying mechanisms by which specific genes lead to diseases. This knowledge may expedite the discovery of new therapeutic targets.
- Identification of New Therapeutic Strategies: AI can assist in drug development, identifying potential candidates that could be effective against specific genetic disorders. This may shorten the time taken for new treatments to become available for patients.
AI's Role in Shaping Future Genetic Technologies
Artificial intelligence is poised to revolutionize genetic technologies. The future holds numerous opportunities where AI methodologies can enhance existing technologies and create novel solutions. Key aspects include:
- Automation of Genomic Data Processing: Automation can streamline processes in sequencing and analysis, drastically reducing the time and labor costs involved in genetics research.
- Integration with CRISPR Technology: AI tools can enhance CRISPR systems for precise gene editing. They can predict potential off-target effects, making gene editing safer and more efficient.
- Improved Diagnostic Tools: AI-fueled diagnostic tools can provide precise and rapid diagnosis of genetic disorders. These advancements hold potential for clinical settings, allowing for early intervention.
As AI continues to evolve, its role in shaping future genetic technologies will surely evolve too, offering exciting pathways for research and treatment.